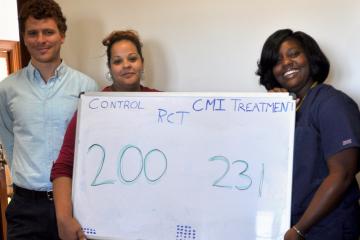
Regression to the mean: What it is and why it matters for impact evaluations
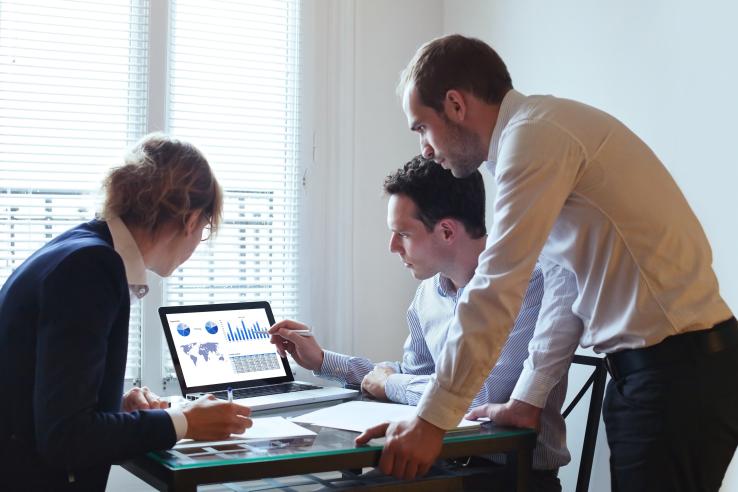
In the late 19th century, researchers studying the heights of individuals and their children observed that both the tallest and shortest parents tended to have children that were closer to average height than themselves. Likewise, the tallest or shortest children tended to have parents that were closer to average height. Researchers have since named this phenomenon regression to the mean: when individuals identified because of an 'extreme value' at one point will tend to trend back toward the average over time, simply because of natural variation in the data. This phenomenon applies broadly. For example, the group of students who received As on one test will tend to have a lower average score on a second test, and the students who received Fs will tend to have a higher score. The explanation is not genetics or test-taking motivation but simply noise, non-systematic variation in the data that means that repeated measurements are imperfectly correlated.
Regression to the mean makes it particularly challenging to estimate program impacts in fields where interventions often start, by design, in response to extreme signals that may not be stable. For example:
- A food-as-medicine program aims to improve blood sugar levels of low-income patients with uncontrolled diabetes by providing fresh food and nutritional education.
- A care management program aims to reduce hospital readmissions for patients who had been in the hospital two or more times in the last six months through care coordination.
- A one-on-one tutoring intervention aims to improve academic outcomes among children who are falling behind grade level.
- An emergency financial assistance program aims to prevent individuals at risk of eviction from losing their homes.
If we observe improvements in an outcome after the intervention, are these improvements a result of the program, or would many of those cases have naturally tended back towards an average outcome without the program?
Regression to the mean and randomized evaluations
Fortunately, randomized evaluations allow researchers to distinguish between regression to the mean and a program’s impact.. Indeed, not only does random assignment eliminate selection bias—ensuring that program participants and the comparison group do not differ systematically at the start of the program—it also allows us to observe what would happen in the absence of the program over time. This eliminates the need to make the assumptions required of a pre-post comparison. In a randomized evaluation, outside factors affect both groups equally, whether it be a pandemic, the progression of time, or a statistical phenomenon like regression to the mean.
In the case of food-as-medicine, J-PAL affiliated researchers conducted a randomized evaluation of a program that provided fresh food and nutritional education to low-income patients with diabetes. In a recent JAMA Internal Medicine paper, researchers found that patients in the intervention group saw improved blood sugar levels at the end of the study. However, patients in the comparison group also improved their blood sugar levels at similar rates. There was no additional improvement for those in the program.
These results are similar to those of a 2020 randomized evaluation studying the Camden Coalition’s care management program for high-need, high-cost patients. The program aimed to reduce hospital readmissions by connecting patients to outpatient services and social support. In this study, both the intervention and comparison groups visited the hospital less over the next six months. There was no added impact on readmissions from participating in the program, despite the program succeeding in connecting patients to outpatient care and medical equipment.
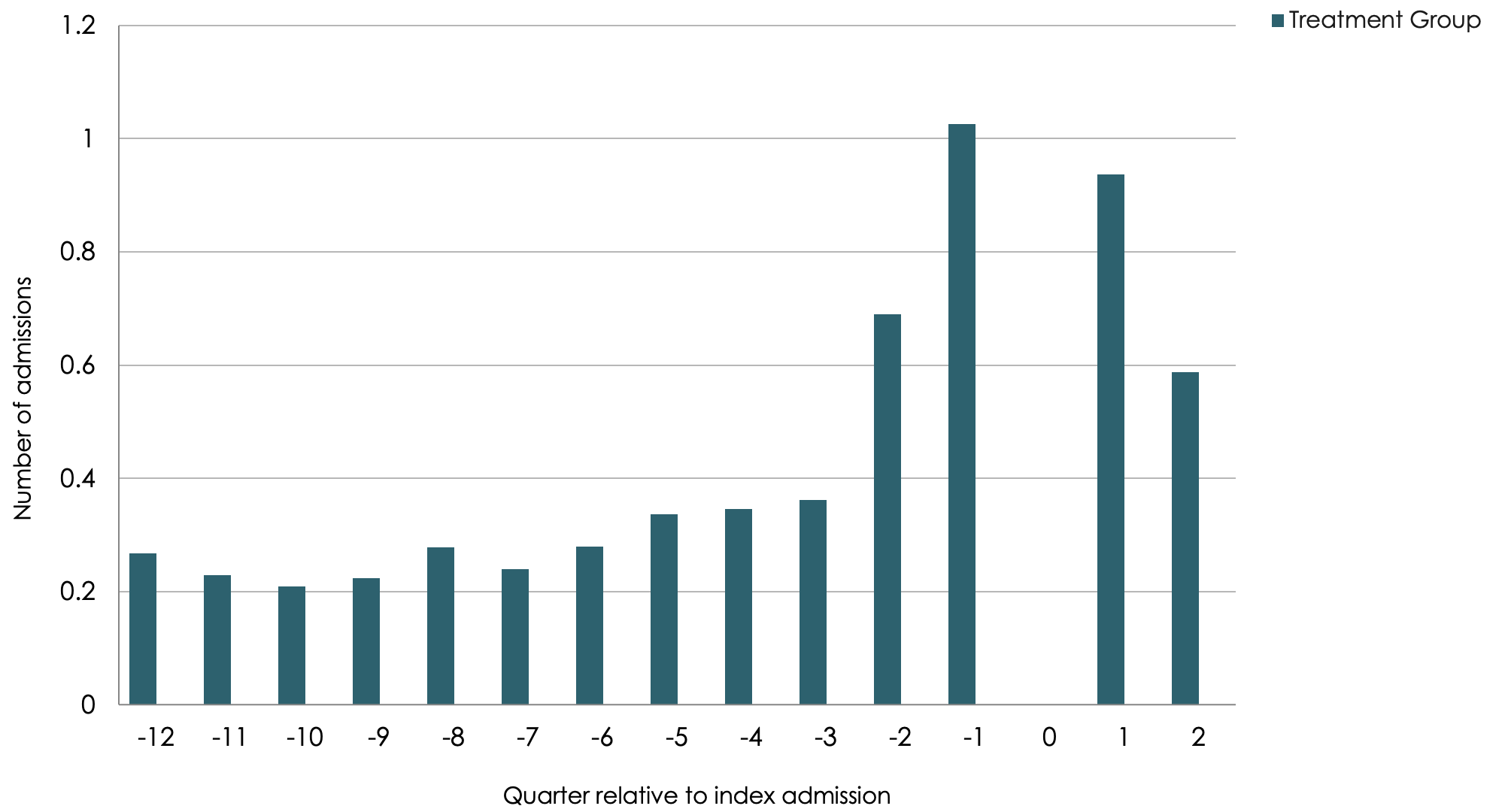
This doesn’t mean that these problems went away—these patients continued to have diabetes and high rates of hospitalizations. However, had researchers only been looking at what happened to those in the program, without a control group for comparison, they would have erroneously concluded that the food-as-medicine and Camden Coalition’s programs were having positive effects.
On the other hand, despite the presence of regression to the mean, many programs implemented in response to extreme signals, such as tutoring and housing stability interventions, have shown positive effects in randomized evaluations. Across 96 randomized evaluations included in J-PAL’s Tutoring Evidence Review, tutoring consistently led to improved learning outcomes and has been a focus of scale up activities in response to Covid-19’s disruption of education. Programs targeting people at risk of eviction—such as Homebase, a comprehensive eviction prevention program—can be effective at reducing time spent in shelters. It would have been difficult to rigorously demonstrate these impacts without randomized evaluations.
Learning from regression to the mean
Being aware of regression to the mean can help researchers and implementing organizations identify priority programs for rigorous evaluation. In the case of high-need, high-cost patients, both previous research and warnings from other practitioners signaled the risk of regression to the mean, but it required a randomized evaluation to rigorously demonstrate the null effect of the program on hospital readmissions.
As programs often only have access to pre-post data from their participants before launching a randomized evaluation, researchers should be aware that rigorously evaluating programs in these contexts may end up revealing that promising interventions are not as effective as they seemed. Researchers should prepare implementing partners accordingly and assess their willingness to learn about their programs, regardless of the outcome, when designing an evaluation and assessing the viability of a research partnership.
Researchers may also want to differentiate between statistical regression to the mean and alternative explanations for improvements in the comparison group, such as behavior change in response to initial measurements or being in a research study. To do so, researchers should consider additional study arms, such as a supplemental comparison group that is less involved in study procedures, or replicating the evaluation in a different setting with fewer wraparound services. For example, researchers speculated in the food-as-medicine program that access to care within an integrated health care system may have allowed all participants to reduce their blood sugar levels.
For researchers aiming to bolster the well-being of people facing extreme circumstances, being aware of regression to the mean and how it affects impact evaluations is key to generating rigorous, actionable evidence.
Related Content
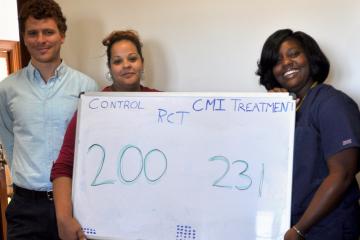
Evaluating the Camden Core Model: How a research partnership between the Camden Coalition and J-PAL North America was built

Did a high-profile program really slash hospital spending? Or was it a cautionary tale of 'regression to the mean'?
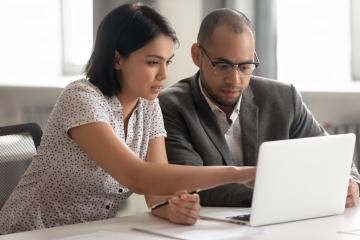