Integrating qualitative methods into randomized evaluations
Summary
From ideation and early research design to uncovering causal mechanisms or details about unexpected outcomes, careful qualitative work can be an important complement to the quantitative portion of an RCT. This resource illustrates what can be learned from qualitative data analysis at different stages of the RCT project cycle. Readers interested in learning more about implementing different qualitative methods may refer to the corresponding resource on implementing qualitative methods in the field.
Qualitative methods at different stages of the project cycle
Qualitative methods add distinct value at each stage of the project lifecycle.
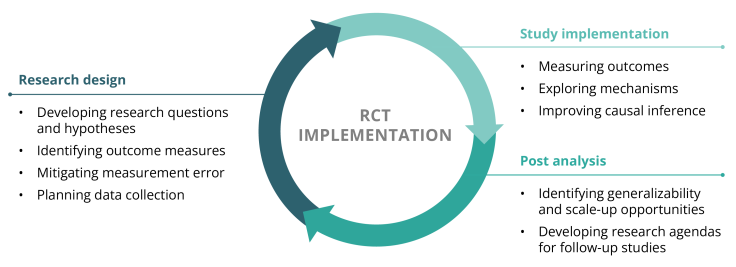
Research design
At the research design stage, qualitative tools can be used to identify research questions, treatment content, and outcome measures that are appropriate and relevant to the study context. Qualitative methods commonly used for this purpose include in-depth interviews, focus group discussions, and observations through site visits.
Developing research questions and hypotheses
Research questions, hypotheses, and empirical strategies can be refined through an iterative process that incorporates qualitative data. At the outset of designing a randomized evaluation, it is critical to understand the local social, political, and economic conditions that can affect how (or whether) an experiment can be run as well as the likelihood of a treatment having its hypothesized effect. To help identify an appropriate research question and generate realistic hypotheses, researchers may want to collect qualitative data from relevant stakeholders to understand:
- Which approaches have been considered and implemented in the past.
- Any obstacles that might inhibit certain programs from being effective.
- Types of programs that appear to be more promising based on the perceptions of implementers and participants.
For example, in research by Markus Goldstein and Chris Udry (2002) about household organization, land tenure, and soil fertility, open-ended and extensive discussions with farmers were important to clarify the incentives confronting individuals as they managed their land (Udry 2003). In this study, qualitative data helped refine preliminary hypotheses and adjust data collection procedures to the local context. Pérez Bentancur and Tiscornia (2022) suggest a systematic approach to iteration based on their study of public opinion support for punitive policing practices in Uruguay, which combines a survey experiment with qualitative fieldwork, including direct observation and interviews. Their approach includes the following steps (Pérez Bentancur and Tiscornia 2022):
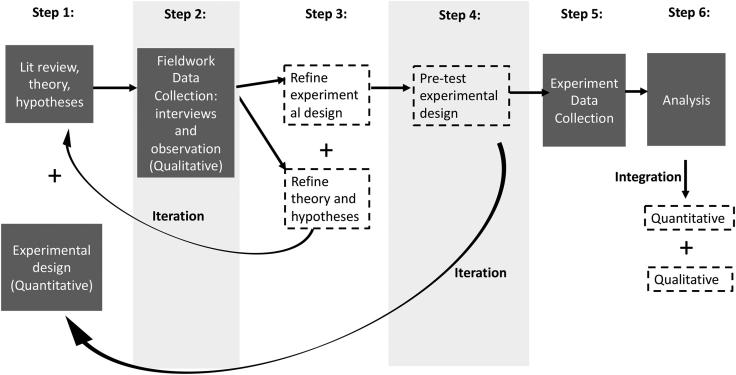
Identifying outcome measures
Qualitative methods can be used to inform decisions about which outcomes are most appropriate and feasible to measure for a given treatment. For certain kinds of studies, relevant outcomes may be fairly obvious, such as voting (i.e., did the respondent vote in the most recent election) for an intervention aimed at increasing voter turnout. However, other kinds of studies may have a wider range of potentially relevant outcomes to choose from. Data collected from key stakeholders through interviews or focus group discussions can help researchers prioritize among different potential outcome measures, which could have implications for both data collection strategies and statistical analysis (e.g., whether to index related outcome measures, correct standard errors for multiple hypothesis testing, or focus on a smaller number of conceptually distinct targeted outcomes).
For example, a study evaluating the effects of a women’s empowerment intervention on the substantive representation of women’s interests could evaluate women’s representation in a variety of ways: Do local leaders know what women’s preferences are? Do local leaders integrate women’s preferences into government planning documents, government budgets, or regulations? Selecting the most appropriate primary outcomes to measure should depend on a number of factors, such as:
- How are government decisions made in this context? For example, non-binding government plans may afford more symbolic and less substantive representation compared to binding budgets that actually direct the distribution of state resources.
- Which types of representation are important to community members? For example, women in the study sample might care less about whether their ideas are talked about by leaders as long as their ideas are included in the budget.
Recent work by Seema Jayachandran combines the use of qualitative methods and machine learning to develop a systematic approach to identifying survey measures of abstract constructs. Jayachandran, together with an interdisciplinary research team, conducted in-depth interviews with 209 married women in North India to validate quantitative measures of women's empowerment. Qualitative data was used to first create a benchmark measure; machine learning algorithms were then applied to identify survey questions that best predicted women’s empowerment when compared to the benchmark.
Mitigating measurement error
Qualitative methods can also be used to reduce the likelihood of measurement error. For example, one might be concerned that respondents will interpret a survey question differently than the researcher intended or that respondents might interpret the same question differently from one another. This can be a challenge for even seemingly straightforward questions like, “Have you contacted any local officials about issues accessing public services in the past month?” In this example, respondents may have context-specific interpretations of who are local officials and what counts as contact (e.g., some respondents might answer “no” if they had an informal conversation on this topic with a neighborhood leader, while others might answer “yes” based on the same experience).
By asking open-ended questions about respondents’ interpretations of survey questions during piloting, you can gauge whether their understanding of the question is as intended and whether they found any questions or response options ambiguous or confusing. Based on the qualitative information from these open response questions, researchers can refine the survey questions to be used in the baseline, midline, and/or endline surveys. Returning to the previous example, based on qualitative information about respondents’ understandings of who counts as a ‘local official’ and what counts as ‘contact,’ researchers may decide to make the question more specific by asking about certain types of local officials and/or certain types of communication. For more guidance on survey design, see our research resource and repository of measurement and survey design resources.
Planning data collection logistics
Qualitative data can help you figure out how to engage hard-to-reach populations and understand security challenges. For example, prior to launching an RCT, Innovations for Poverty Action (IPA) conducted focus groups, semi-structured interviews, and rapid ethnographic assessments with Venezuelan migrants in Colombia to inform outreach strategies for providing temporary regular immigration status (IPA 2022).1 In addition to experiencing high socioeconomic vulnerability, migrants mistrusted regularization institutions and feared arrest or deportation. This qualitative data collection helped researchers better understand the social dynamics of the target population for the randomized intervention that would follow (e.g., how migrants share information with one another). Based on this deeper understanding of Venezuelan migrants’ experiences, the researchers refined the sampling and recruitment strategies and the treatment design for the RCT.
Study implementation
During study implementation, qualitative methods can be used to collect richer data on key quantitative outcomes, uncover causal mechanisms, and identify threats to causal inference. Qualitative methods commonly used for this purpose include in-depth interviews, focus group discussions, and observations through site visits.
Measuring outcomes
Qualitative data can also be used to measure outcomes in ways that provide more nuance than quantitative measures alone. One approach is to collect alternative forms of data on outcomes. For example, audio or video recordings of participants, observations of program delivery, or other program documentation can be collected and analyzed qualitatively or quantitatively alongside quantitative findings.
Another approach is to add open-ended questions to survey instruments. This allows respondents to share more detailed information, enabling researchers to draw clearer distinctions between respondents’ experiences. Respondents’ explanations for their answers could then lead the researchers to draw different, and potentially more accurate, conclusions about the outcome or processes of interest in the study.
For example, a researcher may be interested in how a women’s empowerment intervention affects the quantity and quality of women’s political participation. How often women participate can be measured quantitatively with survey data, voting records, or public meeting attendance data. However, the quality of women’s political participation is harder to measure with quantitative instruments. Suppose the researcher is interested in how persuasive women’s arguments are in public forums. This could be measured through meeting transcripts or video recordings, whereby the research team may analyze the content and tone of women’s remarks and the remarks of other participants (e.g., Parthasarathy, Rao & Palaniswamy 2017). Natural language processing methods can be used to generate such measures from large samples of texts.
Likewise, collecting qualitative data alongside a quantitative outcome measure can allow for a more comprehensive assessment of the outcome. For example, two respondents may answer an attitudinal Likert scale question identically but provide different explanations for their answer in an open-ended question. Such qualitative data could be incorporated to rank differences among otherwise identical dichotomous or ordinal values (Glynn and Ichino 2014), or to help understand mechanisms that may explain how the treatment caused different outcomes.
Exploring mechanisms
Causal mechanisms refer to the pathways through which a treatment affects an outcome of interest. Qualitative research methods can help unpack the mechanisms behind an intervention's impact, which can corroborate hypothesized theories of change or provide possible explanations for surprising or null results. One way of unpacking these mechanisms within the implementation phase is to use qualitative methods to explore the validity of a program’s theory of change (Kabeer 2019). Qualitative methods can be used in implementation monitoring to help determine the quality and fidelity of a program’s delivery, which can uncover mediators or barriers to expected outcomes. This can either corroborate evaluation findings or identify areas in the causal pathway which fail to perform as anticipated due to improper implementation or incorrect assumptions.
Null or unexpected results
Qualitative analysis can be especially useful in cases of surprising or null results from statistical analysis. In such cases, researchers may want to measure additional covariates and test for heterogeneous treatment effects to understand whether treatment effects were moderated by certain factors (e.g., the treatment may have had positive effects for one subgroup and negative effects for another subgroup, resulting in a null average treatment effect). Since it may be difficult to predict unexpected outcomes from the outset, designing quantitative instruments to capture these types of effects ex ante can be challenging. Collecting high quality qualitative data from the start of the intervention can help provide some explanation of null or unexpected results when they do appear.
For example, Rao, Ananthpur and Malik (2017) conducted ethnography with 10% of participants in an RCT to help explain null quantitative results. Their qualitative data collection was more in-depth and open-ended than the close-ended surveys used for the quantitative analysis. As such, they were able to reveal specific mechanisms for the intervention’s failure and identify subtle treatment effects that would not have been detected through statistical analyses. In particular, the qualitative analysis captured context-specific processes, unintended consequences, and longer-run effects that were unobservable in the endline survey data. For example, the qualitative analysis revealed how the intervention—a citizenship training and facilitation program in rural India—raised expectations about participatory processes in the community that led to frustration with subsequent visits from NGOs that were less effective as trainers and counterparts. Qualitative data provided evidence of heterogeneity in the quality of treatment (based on the variable effectiveness of different facilitators) and delayed effects, manifesting after facilitators concluded their involvement with village councils.
Improving causal inference
Qualitative data can help characterize non-compliance, attrition, and spillovers, which can all lead to biased estimates of key outcomes. Qualitative data may help researchers understand different motivations for non-compliance and attrition, which could help sign the bias (i.e., upward, downward, or attenuation bias), or to help measure the prevalence and nature of spillovers. For more information on threats to causal inference, see our Data analysis resource.
- Non-compliance: Qualitative data can provide evidence about the number of defiers and whether they are affected by treatment through the same mechanisms as compliers (Seawright 2016). This is important because the existence of defiers in the sample will bias intent-to-treat (ITT) estimates even if they are evenly distributed across treatment arms. For example, if there are fewer defiers than compliers, defiers will bias the ITT estimate towards zero. Seawright (2016) recommends choosing noncompliant cases from the group with the highest compliance rate when searching for potential defiers, and then assessing “the character of the causal link between treatment assignment and actual treatment received among selected, noncompliant cases.” This assessment can be done with qualitative methods aimed at determining whether a non-compliant subject is non-compliant because they are inattentive or because they are suspicious of the intervention (the former suggesting that the subject is a never-taker and the latter suggesting that the subject is a defier).
- Attrition: Like non-compliance, attrition can also be a threat to inference in an RCT, and qualitative data can help characterize the nature of the threat. If the control group has a higher rate of attrition than the treatment group, estimated treatment effects may be biased. Collecting qualitative data about the subjects’ motivation for withdrawal can help the researcher estimate the direction of bias caused by attrition.
- Spillovers: While certain types of spillovers can be measured and modeled quantitatively (e.g., geographic spillovers), others might only be revealed through qualitative information. Researchers might be able to observe this type of spillover through qualitative interviews or observation of subjects’ routines and activities. After learning through qualitative methods where or how spillovers are occurring, researchers might then collect quantitative data and model the likelihood of spillovers accordingly in the statistical analysis.
Post-analysis stage
In the post-analysis stage, qualitative data can be used to assess the generalizability of findings, determine whether an intervention is appropriate for scaling, or guide next steps in terms of setting a possible research agenda for follow-up studies. Qualitative methods commonly used for this purpose include in-depth interviews and focus groups discussions.
Identifying generalizability and scale-up opportunities
Qualitative methods can be used to further situate post-analysis results within the research context, shedding light on aspects internal to a study as well as the wider social and political setting. This can help determine how generalizable study findings are to other groups that may benefit from the intervention as well as the scalability of the program. Contextualizing findings can be particularly useful when interpreting heterogeneous effects, where qualitative data can help identify contextual mechanisms behind differences in outcomes among sub-groups. This can help researchers and policymakers understand why an intervention was effective, which elements were crucial to the treatment’s success, and how the intervention could be adapted for scale-up or implementation in a different context.
Developing research agendas for follow-up studies
The knowledge generated from qualitative analysis can help better inform next steps in terms of future research, program design, and policy decisions around specific interventions. Uncovering mechanisms that underlie an intervention's success or failure can guide future program design to address contextual challenges and improve program delivery. In the case of heterogeneous effects, insights from qualitative analysis can help guide decisions around expanding or modifying programs to encourage more equitable outcomes.
Considerations for using qualitative methods
Concerns about bias
The use of qualitative methods in experimental research may elicit questions around maintaining rigor when integrating these methodologies as well as the feasibility of carrying out both quantitative and qualitative activities within the same study. Maintaining researcher objectivity and capturing representativeness can be topics of particular interest to quantitative researchers when it comes to using qualitative methods. Morse (2015) provides a detailed definition of rigor in qualitative research and gives brief overviews of different strategies to address components of rigor.
Researcher objectivity
A common concern around researcher objectivity is that qualitative research relies too heavily on researchers’ interpretive judgment due to the open-ended nature of the methodology. To address this concern, researchers can employ practices to ensure transparency throughout the research process. A few of these practices include:
- Thorough documentation of the research protocols and procedures, including interviewer characteristics, sampling frame, non-response rates and characteristics, saturation threshold, ICR standard, etc. (Tong, Sainsbury, and Craig 2007)
- Disclosing idiosyncratic features of the research design when reporting results
- Working in interdisciplinary teams
- Triangulation of findings with other sources of data (Starr 2004)
Representativeness
Concerns around representativeness often stem from the small sample sizes typically used in qualitative research. Though representativeness is not an issue limited to qualitative research, there are tradeoffs researchers must contend with in terms of breadth and depth when selecting the number of cases to investigate qualitatively within a study sample. Since time and budget constraints may be prohibitive to gathering in-depth qualitative data from a large sample, purposive sampling may allow researchers to cover a variety of cases with characteristics of interest that may further explain certain outcomes. Alternatively, Paluck (2010) proposes collaboration as a way to allow sampling of a larger number of cases, whereby each researcher takes a portion of the sample to collect qualitative data from, with some overlapping cases to serve as quality checks.
Forming interdisciplinary research teams
It is also important for members of the research team, from any discipline, who are involved in qualitative data collection, to be trained in qualitative research to ensure qualitative data is collected rigorously and ethically. Team members with expertise in qualitative methods can also be well-placed to lead community engagement efforts and build trust with the study sample. Establishing an interdisciplinary research team can be an effective way to ensure that there is sufficient expertise in qualitative methods among a study’s team members. Input from researchers with diverse perspectives can also provide valuable insights to the local context in which the intervention is taking place, which can help to identify key variables and mechanisms that may be difficult to measure with quantitative methods alone.
Planning ahead for a mixed methods approach
Planning for a mixed methods approach to a randomized study from the outset can help researchers mold more effective and sound research strategies for both quantitative and qualitative approaches. Integrating qualitative methods into an RCT often has implications for budgets and personnel, as these methods often require additional equipment, fieldwork, staff time, and specialized expertise. It is important to account for the significant amount of time that can be involved when collecting and analyzing qualitative data.
In light of these additional requirements, it’s best to consider qualitative activities early in the course of study planning and to budget for additional costs in funding and grant proposals. This can allow researchers more time to budget and plan for different quantitative and qualitative data collection phases and analyses (Catallo et. al. 2013).
A growing number of researchers who conduct randomized evaluations have already begun systematically integrating qualitative methods into the design of their experiments, including qualitative interviews and ethnography (e.g., Blattman et al. 2023; Paluck 2010; Rao, Ananthpur and Malik 2017; Gazeaud, Mvukiyehe, and Sterck 2023; Londoño-Vélez and Querubín 2022). We hope this resource will help more research teams rigorously incorporate qualitative methods into various stages of RCT research, enabling more contextually-informed research designs and deeper understandings of research results.
We thank David Torres Leon, Nicolas Romero Bejarano, William Parienté, Sarah Kopper, Diana Horvath, and JC Hodges for helpful comments. All errors are our own.
Additional Resources
edited by Norman K. Denzin, Yvonna S. Lincoln. 2011. “The SAGE Handbook of Qualitative Research.” Thousand Oaks :SAGE.
Seawright, J. (2021). What Can Multi-Method Research Add to Experiments? In Druckman, J. N., & Green, D. P. (Eds.), Advances in Experimental Political Science. Cambridge, UK: Cambridge University Press. https://doi.org/10.1017/9781108777919.026.
References
Blattman, Christopher, Gustavo Duncan, Santiago Tobón, and Benjamin Lessing. 2023. “Gang Rule: Understanding and Countering Criminal Governance.”
Catallo, Cristina, Susan M. Jack, Donna Ciliska, and Harriet L. MacMillan. 2013. “Mixing a Grounded Theory Approach with a Randomized Controlled Trial Related to Intimate Partner Violence: What Challenges Arise for Mixed Methods Research?” Nursing Research and Practice 2013 (1): 798213. https://doi.org/10.1155/2013/798213.
Gazeaud, Jules, Eric Mvukiyehe, and Olivier Sterck. 2023. “Cash Transfers and Migration: Theory and Evidence from a Randomized Controlled Trial.” The Review of Economics and Statistics 105 (1): 143–57. https://doi.org/10.1162/rest_a_01041.
Glynn, Adam N., and Nahomi Ichino. 2015. “Using Qualitative Information to Improve Causal Inference.” American Journal of Political Science 59 (4): 1055–71. https://doi.org/10.1111/ajps.12154.
Goldstein, Markus, and Christopher Udry. 2008. “The Profits of Power: Land Rights and Agricultural Investment in Ghana.” Journal of Political Economy 116 (6): 981–1022. https://doi.org/10.1086/595561.
IPA. 2022. “The Role of Community Leaders in the Regularization Process of Venezuelan Migrants in Colombia.” IPA Policy Brief.
Kabeer, Naila. 2019. “Randomized Control Trials and Qualitative Evaluations of a Multifaceted Programme for Women in Extreme Poverty: Empirical Findings and Methodological Reflections.” Journal of Human Development and Capabilities 20 (2): 197–217. https://doi.org/10.1080/19452829.2018.1536696.
Londoño-Vélez, Juliana, and Pablo Querubín. 2022. “The Impact of Emergency Cash Assistance in a Pandemic: Experimental Evidence from Colombia.” The Review of Economics and Statistics 104 (1): 157–65. https://doi.org/10.1162/rest_a_01043.
Morse, Janice M. 2015. “Critical Analysis of Strategies for Determining Rigor in Qualitative Inquiry.” Qualitative Health Research 25 (9): 1212–22. https://doi.org/10.1177/1049732315588501.
Parthasarathy, Ramya, Vijayendra Rao, and Nethra Palaniswamy. 2017. “Deliberative Inequality : A Text-as-Data Study of Tamil Nadu’s Village Assemblies.” Text/HTML. World Bank. Accessed August 6, 2024.
Pérez Bentancur, Verónica, and Lucía Tiscornia. 2022. “Iteration in Mixed-Methods Research Designs Combining Experiments and Fieldwork,.” Sociological Methods & Research 53 (2): 729–59. https://doi.org/10.1177/00491241221082595.
Rao, Vijayendra, Kripa Ananthpur, and Kabir Malik. 2017. “The Anatomy of Failure: An Ethnography of a Randomized Trial to Deepen Democracy in Rural India.” World Development 99 (November):481–97. https://doi.org/10.1016/j.worlddev.2017.05.037.
Sangaramoorthy, Thurka, and Karen A. Kroeger. 2020. “Rapid Ethnographic Assessments: A Practical Approach and Toolkit For Collaborative Community Research.” Routledge. https://doi.org/10.4324/9780429286650.
Seawright, Jason. 2016. “Multi-Method Social Science: Combining Qualitative and Quantitative Tools.” Strategies for Social Inquiry. Cambridge: Cambridge University Press. https://doi.org/10.1017/CBO9781316160831.
Starr, Martha A. 2014. “Qualitative and Mixed-Methods Research in Economics: Surprising Growth, Promising Future.” Journal of Economic Surveys 28 (2): 238–64. https://doi.org/10.1111/joes.12004.
Tong, A., P. Sainsbury, and J. Craig. 2007. “Consolidated Criteria for Reporting Qualitative Research (COREQ): A 32-Item Checklist for Interviews and Focus Groups.” International Journal for Quality in Health Care 19 (6): 349–57. https://doi.org/10.1093/intqhc/mzm042.
Udry, Christopher. 2003. “Fieldwork, Economic Theory, and Research on Institutions in Developing Countries.” American Economic Review 93 (2): 107–11. https://doi.org/10.1257/000282803321946895.