Artificial intelligence for social good
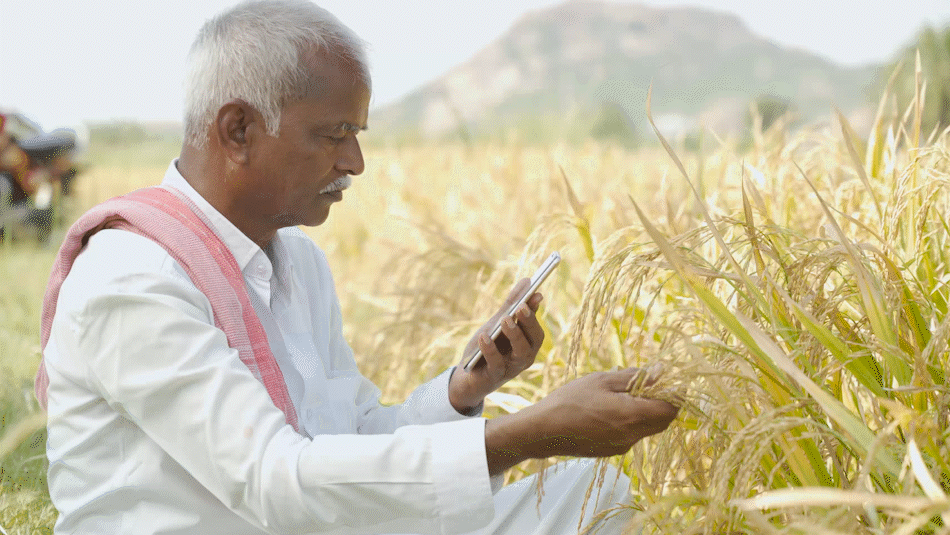
Our vision
J-PAL is helping shape the direction of AI in the social sector and the fight against poverty. By learning from past evaluations and drawing on insights from new evaluations, we aim to maximize its benefits while mitigating potentially dangerous risks.
Channels for AI impact in the social sector
AI has the potential to transform our lives. Like the internet or electricity, AI is a general purpose technology that spans sectors. It is also widely accessible, has low marginal cost of implementation, is in reach of anyone with a smartphone, and is constantly improving.
We believe that AI has massive potential in fighting poverty through at least the following five channels:
1. Improved targeting and needs prediction
Social programs use a variety of tools to identify who the participants should be in programs like targeted alerts or job skilling / entrepreneurship training. The problem is that these tools, including surveys and poverty lines, can be blunt, expensive, and ineffective. AI can enable us to leverage tremendous amounts of data to identify who is most in need of support, or most likely to succeed by participating in that program.
- For example, in India, low-income households in remote areas are particularly vulnerable to floods, which can disrupt or destroy livelihoods and cause severe financial damages. It may not be clear which communities require the most support, and the resources and capacity to provide support are often in short supply. In partnership with Google, J-PAL affiliated researchers are evaluating a flood forecasting AI model. The model predicts flood locations and water levels, sending alerts to Android smartphones in affected areas. These warnings help at-risk households prepare and evacuate and enable the government to preemptively direct resources to improve resilience in rural areas.
2. Increasing access to services
Most social programs and organizations do not have enough capacity to reach everybody who needs them. AI-powered tools can bridge the gap between existing supply of service providers and the underserved population, ensuring that essential services are available to more people, especially in remote or resource-constrained areas.
- For example, there are not enough agricultural extension workers to visit every smallholder farmer or bankers to advertise small-business loans to every entrepreneur. J-PAL researchers are currently evaluating ongoing advances in machine learning that enable any farmer with access to a smartphone to take a picture of a crop and receive instant advice on how to improve their yields. AI could significantly level the playing field and reduce key obstacles for those who would most benefit from these services.
3. Maximizing the effectiveness of frontline service providers
Frontline workers typically have the skills necessary to carry out basic duties, but lack the advanced knowledge, skills or training needed to handle more complex, but needed challenges. AI can enhance the decision-making capabilities of frontline workers and enable them to perform more high-value work.
- For example, community health providers (CHPs) are a crucial source of basic care in lower- and middle-income countries, where there aren’t enough doctors. But a CHP may not have a formal degree in medicine or a group of experienced peers with whom they can consult on a medical case. A properly trained model could provide diagnostic assistance and evidence-based treatment protocols, thus leading to better health outcomes. J-PAL researchers are proposing the development of an automated screening tool that uses machine learning to predict the results of expensive diagnostic tests from the results of lower-cost mobile tests that can be performed at a patient’s home.
4. Reducing biases and ensuring fairness
It’s true that algorithms can carry biases. But algorithms are not inherently biased, and we can engineer these issues out. When programmed correctly, AI can even help identify and mitigate biases in government and social programs, ensuring fairer distribution of services.
- For example, evidence from J-PAL researchers has shown that human-made systems, such as hiring processes, can be rife with bias. On the other hand, algorithms designed to screen candidates based on their upside potential rather than simply favoring those with attractive resumes can be used to address these biases and drive more equitable outcomes.
5. Boosting governmental capacity
Just as the fight against climate change and poverty is in need of a huge increase in resources, governments worldwide are staring at unsustainable fiscal deficits, thanks in part due to pandemic era growth shocks and high spending on support programs. This requires increasing compliance to existing tax rules. In parallel, AI can take something that was previously tedious and expensive, turn it into an instantaneous and less costly process, freeing up capacity for tasks that humans are better suited for and reducing the need to expand the size of governments or NGOs.
- For example, better identification of fraud and non-compliance in paying taxes currently requires a lot of tedious work. But J-PAL affiliated researchers working in India found that machine learning techniques can identify potential tax evaders, potentially widening the tax base massively. Human workers can then verify these cases with in-person follow ups.
A framework for effective AI adoption for social good
Currently, causal evidence on where and how AI can change society is scarce. Without such evidence, companies, social innovators, and regulators lack the information to maximize the benefits of AI while protecting against its downsides.
As this technical revolution unfolds in real time, we have a responsibility to rigorously study how these technologies can help–or harm–the well-being of people, particularly people who experience poverty.
J-PAL has a long history of using randomized evaluations to test programs in sectors like education and health. We can draw insights from decades of rigorous research to understand which social programs AI can be implemented in for the most benefit. We can also apply lessons from past studies on successful technology integration to ensure AI solutions work in real-world programs.
From there, there is a need to conduct new evaluations of AI applications across sectors to understand what does and does not work, and why. As we learn more about which AI-infused interventions work to improve people’s lives, we will disseminate lessons from evaluations and help policymakers scale up promising innovations.
Launching an AI for Social Good Initiative
We are seeking funding partners to help launch a new research fund, modeled on J-PAL’s successful initiative model. To ensure that AI implementation maximizes social benefits and minimizes risks, the new initiative will focus on the following goals:
-
Identifying and supporting innovative organizations to use AI to advance social good.
-
Funding rigorous research to measure the causal impact of their AI solutions on social outcomes in priority areas.
-
Scaling and applying socially-beneficial AI solutions based on learnings from the research.
-
Building capacity of governments, social enterprises and NGOs to better incorporate and evaluate AI for social good.
While we are interested in AI's application in every sector, this initiative will initially focus on five priority sectors: (1) economic opportunity and jobs, (2) health, (3) climate change, (4) education, and (5) finance. Some urgent policy questions that we aim to address with this initiative are listed below.
If you are interested in working with J-PAL toward these goals or learning more, we want to hear from you. Please contact our team.
Urgent policy questions
Economic opportunity and jobs
- Skills and training
- Can AI tools improve training and upskilling for job seekers and workers? Can AI tools make training more accessible and customized?
- Do soft skills help workers and managers better adapt to new technology? Do large language model (LLM) tools augment soft skills or substitute for them?
- Workforce organization
- How do LLM tools affect manager-employee relationships?
- What kinds of LLM tools are better suited for highly technically skilled workers versus lower skilled workers?
- What are the best applications of AI to reduce discrimination and bias in the workplace and in hiring? How should policymakers regulate the use of AI in hiring?
- How can firms build new teams or reconfigure teams around AI tools in a way that increases productivity?
- Job search and matching
- How can AI and other technologies enhance matching between employers and job seekers, particularly on online job search platforms? Can AI-enabled tools help job seekers overcome information barriers to finding a job?
- Can AI-enabled tools encourage increased or broader job search activities, and how does this influence employment and earnings?
- SME profitability and sustainability
- What are the best models of AI interventions to increase the profitability, resilience, and sustainability of micro and small enterprises, which provide the majority of employment worldwide and economic opportunities for women in particular? How can we encourage small businesses to take up beneficial AI tools?
- How can AI-based service providers design online platforms, government procurement systems etc. to both be equitable and encourage the entry of new entrepreneurs, women, and underrepresented groups?
- Can AI help identify high-growth-potential entrepreneurs to accelerate job creation in low and middle-income countries? Can microfinance and other credit providers improve credit access to small businesses and entrepreneurs using AI?
- Welfare effects
- Are there displacement effects from AI and how do they vary across sectors/industries/labor markets and by gender, race and ethnicity, and socioeconomic status? What will be the impacts of AI on offshoring jobs?
- What are the impacts of AI on differently skilled staff? Which skill/expertise levels are AI more useful for?
Health
- Disease prediction and diagnosis
- How can AI be used to enhance electronic health records and predict who is at risk for various diseases and facilitate early diagnosis, particularly for non-communicable diseases?
- While this is happening to some extent in high-income countries already; how do we integrate such approaches into health service delivery in LMICs?
- How do we best leverage AI to track disease outbreaks to prevent future pandemics?
- Patient behavior
- How can AI be leveraged to nudge patient behavior earlier and tailor recommendations using novel data sources like wearables and smartphones?
- Can AI assisted care or mobile platforms increase adoption of evidence-based health behaviors?
- How can we best leverage AI to improve the targeting of behavior change campaigns and reach individuals with more personalized, relevant health information?
- Health service delivery and equity
- How can AI and other technologies be used to combat discrimination and biases in health service delivery in order to increase equity, particularly for minority groups receiving inadequate care?
- What innovations can reduce discrimination in the AI tools themselves?
- How can we leverage AI to increase the efficiency and effectiveness of health care providers? For example, through AI-enabled diagnostic support, AI-facilitated summaries of physician notes, AI chatbot intake?
- In contexts where there are relatively few highly skilled health care providers, including rural communities, to what extent can AI be leveraged to augment the skills of existing providers within a community to better meet local health care needs?
Climate change and agriculture
- Agricultural and environmental management
- How can AI-tools be leveraged to tailor advice to small-scale farmers to adopt agricultural practices and technologies that enhance their resilience to climate change?
- Can AI-supported forecasting and early warning systems improve how we predict weather, agricultural, or other shocks? Can improved early warning systems enable implementers to act early to support people before a shock occurs? What are the downstream impacts of anticipatory action on behavior, health, and wellbeing?
- Can AI tools combined with sensor data provide low-cost monitoring of nature to facilitate local and/or indigenous land and ocean stewardship, improved payments for ecosystem services contracts, and carbon credits?
- Social and economic resilience
- How do we ensure that people with less access to technology aren’t left behind (or made worse off) by AI and technology solutions?
- Can AI tools be leveraged to provide better information and tailored recommendations to people on local environmental and climate risks like pollution and extreme heat?
Education
- Teacher training
- How can we leverage AI to increase the efficiency and effectiveness of educators? For example, through AI-enabled lesson planning support, AI-facilitated grading, and AI chatbot-assisted tutoring? What are the benefits of the time savings for teachers?
- In contexts where there are relatively few highly skilled educators, including rural communities, to what extent can AI be leveraged to augment the skills of existing teachers to better meet local educational needs?
- Customized teaching and learning
- How can AI-driven educational tools be used to enhance our abilities to assess the right learning level to teach students at, to ensure the best learning outcomes (in line with the Teaching at the Right Level model)?
- How can AI be used to predict which students are at risk of falling behind, facilitating early intervention?
- Increased engagement by students, teachers, or parents
- How can we best leverage AI to make education and skilling more accessible, and reach students with more personalized and engaging learning content?
- How can AI be leveraged to nudge student behavior towards better study habits and tailor learning recommendations using novel data sources like smartphones and tablets? Can AI-assisted learning platforms increase the adoption of evidence-based educational practices?
Finance
- Designing inclusive finance products
- How can AI/ML models assess the creditworthiness of underserved populations where formal credit histories may not exist? What non-traditional data sources can these models use to make these decisions?
- How can AI/ML models be used to assess the specific needs of groups in LMICs to design affordable insurance products? Can AI tools help to automate and speed up insurance claims for customers in LMICs?
- How can AI/ML models be designed to minimize bias against marginalized groups in lending and insurance decisions?
- Can AI/ML models identify borrowers who are more likely to generate higher returns to credit in a scalable and cost-effective way, enabling financial institutions to allocate capital more productively?
- How can AI/ML models be used to improve the targeting of social protection programs and humanitarian assistance?
- Financial literacy
- Can AI-powered tools that provide customized and interactive financial education increase financial literacy levels? How do the impacts of these compare with existing financial literacy programs?
- Can these tools enable individuals to utilize digital financial services and platforms more?
- Consumer protection
- How can AI/ML be used to recognize predatory lending or other harmful practices?
- Can AI/ML models analyze localized data on spending habits, income sources, and financial behavior that can help to create locally relevant policies that maximize positive and minimize negative household outcomes?
Our team
Scientific Advisors
Iqbal Dhaliwal, Global Executive Director, J-PAL, MIT
Sendhil Mullainathan, Professor, MIT and Co-Founder, J-PAL
Staff
Michael Hou, Senior Policy Associate, J-PAL Global
Aditi Namdeo, Director, Strategic Initiatives, J-PAL South Asia
Pritham Raja, AI Lead, J-PAL Global
Jack Cavanagh, Research Manager, J-PAL Global
Other resources
Public talks
Iqbal Dhaliwal, Global Executive Director of J-PAL, discusses AI in the social sector at the 2024 World Economic Forum in Davos.
A panel discussion on “AI: Emerging Challenges and Opportunities” at J-PAL’s 20th anniversary event at MIT in 2023 brought together thought leaders to discuss the likely impacts of evolving AI technology on social and economic issues including productivity, growth, and labor markets.
Panelists included David Autor, Ford Professor of Economics, MIT; Iqbal Dhaliwal, Global Executive Director, J-PAL; Gita Gopinath, First Deputy Managing Director, IMF; Brigitte Hoyer-Gosselink, Director of Product Impact, Google.org; and S. Krishnan, Secretary, India Ministry of Electronics and Information Technology.
J-PAL signs its first dedicated AI MoU in Hyderabad, becoming the research and evaluation partner for the Government of Telangana's AI work. On stage, Iqbal Dhaliwal and Aditi Namdeo greet dignitaries of the Government of Telangana.
Iqbal Dhaliwal joined a panel at the Mastercard Impact Data Summit 2024 on "Pioneering inclusive AI: Expanding the field of impact data and AI" in September 2024. The panel discussed the critical roles of AI and data in driving social impact.
Evaluations of AI and machine learning
Economic opportunity and jobs
- In France, Bruno Crepon and co-authors published a working paper investigating a job recommender system that uses machine learning to improve matches for job seekers.
- In Kenya, David Atkin, Antoinette Schoar, and co-authors partnered with a data annotation company for machine learning algorithms to measure both the impact of their AI 101 training program and this training program plus job referrals on workers.
- In the US, Peter Bergman and co-authors are evaluating the impact of LLM-based coaching for underrepresented job applicants on job search and salary negotiation.
- Also in the US, Peter Bergman is evaluating the impact of an LLM-based chat-agent during the hiring process to assess its impact on demographic disparities in hiring.
Health
- In India, Esther Duflo, Frank Schilbach, co-researchers, and J-PAL South Asia are collaborating with the Government of Tamil Nadu to develop and evaluate a machine learning tool to improve health screening for the elderly: leveraging data from 30,000 diagnostic tests to create less expensive, predictive mobile tests for home use.
- Also in India, J-PAL is in the early stages of collaborating with Wadhwani AI to develop an AI solution for estimating the weight of a baby using smart phone photos and/or video.
- In Kenya, Jessica Cohen, Margaret McConnell, and co-authors partnered with Jacaranda Health to evaluate the impacts of an AI-supported mobile health platform for pregnant and postpartum people on their care-seeking behavior and health outcomes.
- In the US, J-PAL's Health Care Delivery Initiative is funding a project led by Manuela Angelucci and other researchers, who are collaborating with an NGO to develop and evaluate an AI-based mental health mobile app to provide mental health support to immigrants in the US.
Climate change and agriculture
- In Colombia, Santiago Saavedra and co-authors have used AI tools and satellite imagery to detect illegal mines in Colombia to protect people and natural resources.
- In India, J-PAL's King Climate Action Initiative is funding a project led by Rohini Pande and Maulik Jagnani, who are collaborating with Google’s Flood Forecasting initiative to conduct the world’s first randomized evaluation of a flood early warning system paired with community outreach and measuring impacts on behavior, health, and economic wellbeing.
- Also in India, J-PAL's Agriculture Technology Adoption Initiative, Cash Transfers for Child Health Initiative, and King Climate Action Initiative are funding a project led by Kelsey Jack, Seema Jayachandran, Namrata Kala, Rohini Pande, and co-authors who are using machine learning and satellite data to measure the impact of Payment for Ecosystem Services (PES) contracts to avoid crop burning in India (a key contributor to poor air quality).
- In Kenya, J-PAL's Digital Agricultural Innovations and Services Initiative is funding a project led by researchers who are collaborating with Nuru, an AI-enabled mobile application that helps smallholder farmers diagnose and treat plant pests and disease.
- In Peru and Brazil, Alipio Ferreira is using AI to improve satellite enforcement of crop-burning and deforestation regulations in Peru and Brazil. He is working with J-PAL’s Latin America and the Caribbean (LAC) office and Innovations for Poverty Action (IPA) to build an embedded lab within Peru’s Ministry of Environment.
- In the US, Rob Metcalfe is collaborating with real estate companies to evaluate the impact of providing fire risk information to California homebuyers on their housing decisions.
- Also in the US, Michael Greenstone and co-authors are using AI to predict and detect and reduce pollution through improved regulatory enforcement with the US EPA and Colorado.
Education
- In Brazil, Bruno Ferman and co-authors found that an AI-powered educational tool that scored students’ essays enabled teachers to provide more frequent individualized feedback to students, resulting in improved essay scores.
- In Canada, J-PAL's Social Policy Research Initiative is funding a project led by Phil Oreopolous, who is conducting a randomized evaluation to study the impacts of AI-powered tool “Khanmigo,” a personalized tutor designed to support student’s progress with Khan Academy assignments.
- In India, Rocket Learning, an organization at which J-PAL Global Executive Director Iqbal Dhaliwal serves as a board member, is leveraging machine learning and generative AI to create customized content and learning paths for children to help teachers and anganwadi workers develop lessons.
- In South Africa, Cally Ardington is developing an AI-based voice recognition model that performs early-grade reading assessments in African languages.
Finance
- In Egypt, Gharad Bryan, Dean Karlan, and Adam Osman used machine learning in a randomized evaluation to analyze psychometric data on loan borrowers. The model accurately predicted which borrowers would be “high-performing” and “low-performing,” in terms of their returns on a large loan.
Other research
- Daron Acemoglu and David Autor are leading thinkers regarding how AI will impact labor markets. Their paper studied the relationship between AI adoption and job vacancies in the US, and the role of workers' skills when AI is implemented in organizations.
- Jens Ludwig is harnessing data science to help solve social problems and helped establish both the Crime Lab and Education Lab at the University of Chicago. Jens recently co-authored a paper on machine learning for hypothesis generation and another paper on algorithmic bias.
- Christopher Neilson has investigated the impact of several AI interventions like chatbots to boost educational outcomes. He also created ConsiliumBots to leverage AI and data to help policymakers make more informed decisions.
Media coverage
History Already Tells Us the Future of AI
How AI could help rebuild the middle class
Contact us
To learn more about our work, email [email protected].