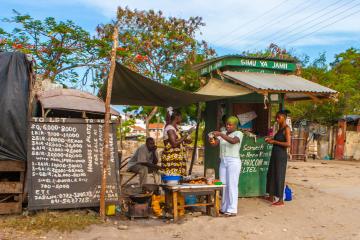
The challenges of targeting social protection programs
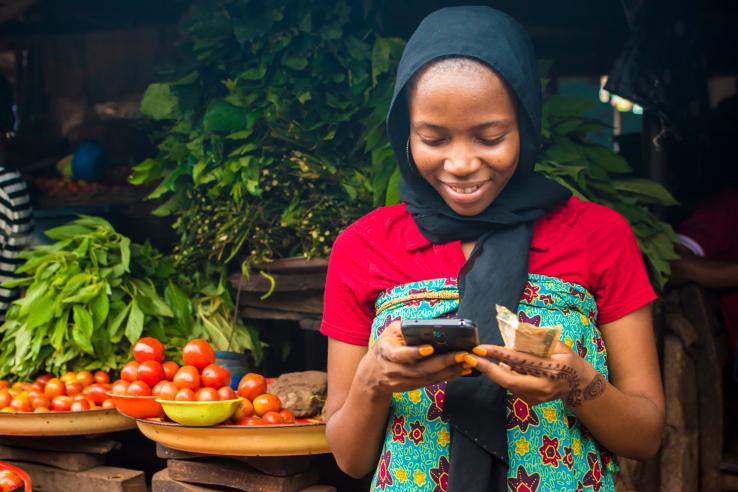
This post was originally published on African Arguments on February 10, 2022, and is part of an ongoing series. Read the other blogs in the series on preparing households for shocks through universal basic income; the benefits and challenges of digital IDs; increasing girls’ enrollment in school; and how different electricity billing systems may impact energy access.
In 2020, when the pandemic began, many governments worldwide undertook the task of channeling emergency support to their most vulnerable citizens. This task, made more difficult by the unprecedented public health restrictions implemented in various settings, was fraught with difficulties. This included ensuring that help reached those who needed it the most, and that precious resources did not go to people who did not need support. Indeed, beyond this recent context, every government in the world faces two major challenges when implementing targeted anti-poverty programs. The first is defining poverty, appropriate thresholds, and measures. The second is identifying benefiting individuals and families themselves.
While the first task involves a range of complex questions on what poverty is and how much income is sufficient to survive or thrive, the second task amounts to a more practical problem: given a poverty level—usually measured as an individual or household living below a certain income level—how can we identify individuals who live under this level, and make sure they receive the benefits they are entitled to?
In advanced economies, thanks to sophisticated tax and data systems, governments can look at who earns what to determine eligibility for social programs. In practice, the problem often is not so simple since a person’s income may not reflect their true earning ability, which is the information one would really need to target social programs effectively. Nevertheless, observing individual incomes can be a big asset in the fight against poverty.
In low- and middle-income countries, however, there is often a large informal sector. Many people tend to rely on several income-generating activities or support from family and friends as opposed to a formal salary. Delivering social benefits to people living in poverty in these contexts can be particularly challenging as governments are unable to observe or measure the income of individuals and small businesses. How can these countries possibly identify the poorest in society in the absence of this data?
Well, there isn’t a single correct answer, but there are several options typically available to governments.
Common approaches to the targeting problem
One popular way of identifying the poor is referred to as proxy means testing (PMT). This method involves collecting data on the assets owned by potential beneficiaries (e.g. how much land they own, the quality of their house materials, whether they own a motorcycle, etc.). Using statistical techniques, this information can then be used to predict the consumption levels of individuals or entire households. Countries such as Bangladesh, Indonesia, Rwanda, Sri Lanka, and Tanzania, along with many others, have used this method to develop targeted social protection programs. However, this methodology often does not measure poverty with sufficient accuracy, and it is expensive to collect all the data required.
Another common targeting method is community-based targeting. This method, unlike PMT, allows local community members to take part in the selection of program beneficiaries. The premise behind this approach is that these community members know a lot more about temporary shocks and personal circumstances (such as illness and unemployment) than the central government and can therefore better assess which households in their communities may be most in need. The weakness of this approach is that it could result in “elite capture”: the local elites involved in this process may select beneficiaries among their close friends and relatives, or even keep the transfers for themselves. Also, community members may not agree on what “poverty” entails and they may have differing beliefs about who is poor in their community, creating imbalances across large areas. This method therefore involves a trade-off between better local information and the risk of elite capture.
In 2012, researchers conducted a randomized evaluation to compare these two targeting methods in the delivery of a cash transfer program in Indonesia. They found that the community had a different understanding of poverty, sometimes leading local community members to allocate benefits to different recipients than those predicted by the PMT. As a result, community-based targeting was associated with higher satisfaction of participants, but targeting “efficiency” (i.e. the extent to which the policy reached the poorest community members, as defined in baseline data) was higher with PMT. This suggests a trade-off. The PMT method might be a better choice for governments seeking to minimize the poverty rate as measured in administrative data. On the other hand, involving local communities in the targeting process may be optimal from the perspective of maximizing citizens’ satisfaction.
The third option used by governments does not require any external assessments of who is poor and who is not. This method is called the self-targeting method. Here, governments establish an incentive system designed so that only the people living in poverty will come forward to claim the benefit. Self-targeting can be an effective way to provide social assistance to people living in poverty. The individuals or families living in poverty may face a lower opportunity cost of time than, for example, those who have formal employment. As a result, these potential beneficiaries may be more likely to want to overcome the ordeals associated with the process of claiming a benefit, such as filing paperwork or walking to a distant administrative site. Self-targeting therefore relies on creating such procedures to incentivize only the relatively less well off beneficiaries to access the benefit. The issue is that barriers created make the transfer relatively less attractive for everyone. Self-targeting therefore does not fundamentally address the risk of exclusion errors— people living in poverty who do not actually access the transfers designed for them.
Rural employment guarantee schemes, which have been implemented in India and in Ethiopia, among other countries, are examples of such an approach. In India, the Mahatma Gandhi National Rural Employment Guarantee Scheme (MGNREGS) guarantees every rural household 100 days of unskilled manual labor per year at the minimum wage: if you show up to work, you are paid. There is mixed evidence on the program’s overall impact on poverty reduction.
A fourth option: When there is little or no data available on the population (i.e. no social registry, no fiscal records) some governments have chosen to target the poorest households through lotteries. Potential participants are first identified, after which the actual beneficiaries are selected through a simple random draw. A good case study is the DRC’s Social Funds Public Work Program, where parts of the World Bank’s Cash for Work program were allocated through lotteries. The problem with this approach is, once again, the potential for exclusion errors: some of the most vulnerable beneficiaries may end up not receiving the benefits.
Targeting in times of Covid-19
When the Covid-19 pandemic began, governments across the world had to act quickly and decisively to provide assistance to the poorest, whose lives and livelihoods would be disproportionately affected. Governments faced the additional challenge of having to identify these groups and providing assistance whilst also avoiding physical interactions as much as possible. In this context, it is perhaps not surprising that many governments chose to move away from conventional targeting methods. Instead, remote targeting solutions leveraging satellite imagery and “big data” (including cell phone data and administrative data), combined with machine learning techniques, gained immense popularity with agencies implementing social programs. Machine learning can help analyze the length of calls, whether received or made, to identify those who may be in need of assistance. For example, wealthier people tend to make longer phone calls, have more contacts, and carry more balance in their mobile money accounts.
The government of Togo, in partnership with Josh Blumenstock et al. utilized satellite imagery to create poverty maps as part of their Covid-19 response program, Novissi*. These maps, which were generated by a machine-learning algorithm which estimated wealth using indicators such as metal roofs and the quality of local roads, were used to prioritize the poorest areas and allow for more granular targeting than could be achieved using the available national surveys. While this is not entirely novel, as Give Directly has long been using satellite pictures to “screen” potential beneficiaries of cash transfers (initially they selected villages with a large proportion of households with thatched roofs), combining machine-learning and phone records with satellite imagery is.
Likewise, the government of Nigeria combined geolocated household survey data with satellite imagery, and other geospatial data, to construct high-resolution poverty maps. These poverty maps were verified using several household surveys. The Bangladeshi government also used phone logs to identify and determine eligibility of those in need.
However, these novel targeting methods are not without their pitfalls, and there are various risks to using consumer data for targeting. Firstly, many of the machine learning models that these data-driven targeting methods rely on still require existing household survey data or government registries to serve as the underlying ‘ground truth’. For instance, as part of Novissi in Togo, researchers used 2018 household survey data to calibrate and validate the algorithms which helped create the poverty maps used to identify the poorest regions in the country. Therefore, many of these experimental approaches have not entirely removed the need for expensive and time-consuming household surveys.
Secondly, inaccuracies in the poverty estimations of these data-driven targeting methods could lead to the exclusion of eligible individuals from receiving much needed assistance. For instance, targeting based on mobile phone data is limited by the penetration of mobile phones. Given that not everyone owns a mobile phone or knows how to operate one in low and middle-income countries, this method could leave some of the most vulnerable excluded from receiving benefits. One could overcome this by including explicit processes for individuals to appeal their eligibility status within the program, but this may be cumbersome.
From an ethical standpoint, these novel targeting methods also raise some questions. The lack of appropriate data protection laws in developing countries is of particular concern. For instance, in 2013, a controversy arose following the disclosure that researchers had obtained the meta-data of nearly 15 million mobile phone subscribers, without their consent, to predict the spread of Malaria in Kenya. In order to ensure the appropriate use of data, it is important that the appropriate safeguards governing data sovereignty, consent, privacy, and transparency are instituted.
As we enter the next phase of life with Covid-19, it will be fascinating to watch how governments, with the help of researchers, continue to adapt their targeting methods to ensure programs reach the very poor. Two years into the pandemic, the need for targeted social assistance remains more acute than ever. While novel data solutions will continue to offer exciting new pathways into improving targeting methods, continuing disruptions to the global economy and vaccine inequity are increasingly putting the world’s most vulnerable at risk. In this context, rigorous research has a key role to play in order to ensure that social safety nets effectively reach their intended beneficiaries.
End notes
* A comprehensive study on the theme is underway and will be published in a few weeks time.
About the series
The Abdul Latif Jameel Poverty Action Lab (J-PAL) and Debating Ideas collaborative blog series—Unpacking the evidence of social programs in sub-Saharan Africa—seeks to contribute evidence-informed perspectives to debates around key questions in the fight against poverty. The material published as part of this blog series is based on J-PAL’s research network and is anchored by more than 260 affiliated researchers at universities around the world who are united in their use of randomized evaluations to identify the most effective approaches to reducing poverty and improving lives.
Related Content
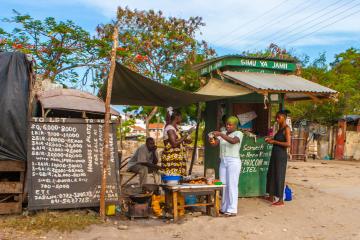
Preparing for shocks through Universal Basic Income: Evidence from Kenya
The rise of mobile money in sub-Saharan Africa: Has this digital technology lived up to its promises?
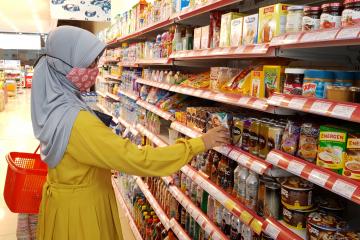