Improving student learning: Impacts by gender
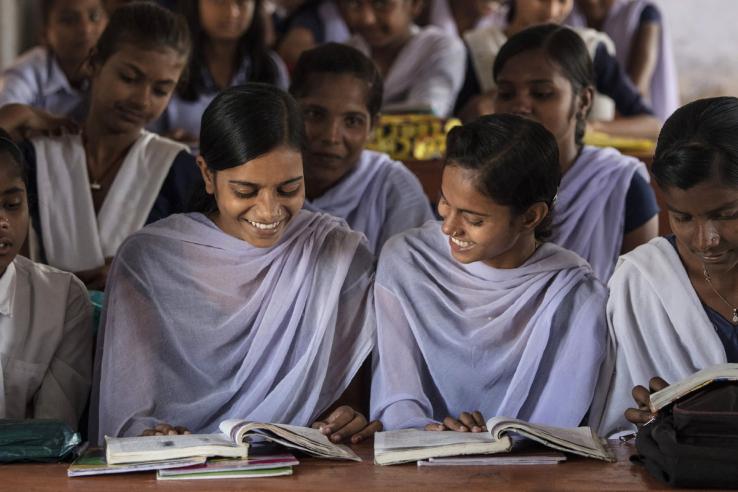
Summary
Over the last two decades, school enrollment has increased dramatically in low- and middle-income countries. However, student learning has lagged behind: even after completing several years of school, millions of students lack basic literacy and numeracy skills [1]. Further, girls, who face additional constraints due to gender norms and gender-based discrimination, might benefit less than boys when they do attend school, which might in turn widen existing gender gaps in learning outcomes.
Globally, girls are twice as likely as boys to never start school and are less likely to complete primary and secondary school. Gender-based differences in learning also exist, but are subject -specific: for example, in most countries, 15-year-old girls, on average, perform better than boys of the same age on reading tests, but worse on science and math tests [1]. Evidence suggests that interventions to increase school enrollment and attendance tend to help the most disadvantaged gender (often girls) most, with some exceptions [2]. Do interventions aimed at improving learning, such as remedial education programs or innovative approaches to teaching, also help the disadvantaged gender more? Further, are gender-neutral interventions sufficient to improve learning among girls, who are often the more vulnerable gender? Or do gendered social norms block gender-neutral programs from benefiting girls, thus exacerbating existing gender inequalities?
Results from 54 studies of programs to improve learning in low- and middle-income countries, all of which reported program impacts by gender, indicate that in a small number of cases, aspects of program implementation, such as preferential treatment afforded to boys by tutors or gender stereotypes, prevented girls from benefiting from programs to the same extent as boys. In other instances, girls benefited more from design features within programs that supported their needs, such as the presence of female role models or the ability to learn in groups with friends. More research is needed to understand how the effects of programs to improve learning vary by gender. Policymakers designing interventions to improve learning may want to account for gender-specific constraints and monitor progress by gender as a best practice, especially in contexts where gender gaps exist in learning outcomes.
One of the key challenges to understanding if and how learning interventions affect girls differently is that studies do not always report program impacts by gender. Hence, randomized evaluations should report gender differences in program impacts more consistently. While a small number of studies that reported program impacts by gender found statistically significant gender differences in impact, a majority did not, which might suggest that learning interventions have had gender-neutral effects or the differences were not large enough to be detected with the given sample sizes. Gender-neutral effects might lead to the persistence or exacerbation of gender gaps in cases where gender inequalities in learning exist before program implementation. Whenever possible, policymakers should consider potential different effects by gender while designing programs to improve learning and to ensure that learning interventions benefit all genders.
Supporting evidence
In some instances, program design features that addressed a gender-specific need, or aspects of implementation that prevented the program from benefiting one gender, may have led to different impacts on boys and girls. In some contexts, program design features to support girls’ learning, such as the presence of female role models or the ability to learn in groups with friends, may have contributed to greater impacts on girls’ test scores. In other instances, aspects of implementation that prevented the program from benefiting one gender, such as preferential treatment afforded to boys or gender stereotypes, may have prevented the program from benefiting girls to the same extent as boys.
Two studies suggest that program design features that support girls’ gender-specific needs might be important to improve learning among girls [3][4]. In Bangladesh, compared to working with random peers, learning in groups with friends increased math test scores by 0.4 standard deviations for disadvantaged girls, who had low test scores before the intervention, but had no significant impacts on boys’ learning outcomes [3]. In Pakistan, a video-based instruction program increased the likelihood of a school visit by parents of boys and boys’ educational aspirations, with no changes for parents of girls or girls themselves, perhaps because 21 of the 22 subject experts in the teaching videos were male [4]. This is consistent with the evidence on gender quotas from India, which suggests that the presence of role models can improve girls’ aspirations and parents’ aspirations for girls [5].
Two studies indicate that some aspects of program implementation prevented programs from benefiting one gender [6][7]. In Peru, an innovative program to teach science at public primary schools using LEGO kits improved test scores for boys but not for girls, accentuating gender inequalities. Anecdotally, during classroom visits, researchers observed that the LEGO kits, which were limited in number (one per class), may have been monopolized by boys [6]. In another study in Peru, the effects of a targeted remedial education program were entirely driven by boys and created a gender gap in test scores [7]. Boys and girls were equally likely to attend tutoring sessions and had comparable levels of achievement in tests before program implementation. Researchers conjectured that gender differences in impact could have been a result of preferential treatment afforded to boys by tutors or boys’ greater engagement in small group tutorials.
Further, one study indicates that interventions that address gender-specific barriers to education attainment faced by girls can benefit girls more and shrink existing gender gaps in learning [8]. In Afghanistan, creating schools in areas without access to formal government schools increased girls’ school enrollment and test scores [8]. In under a year , the creation of schools increased test scores by 0.66 standard deviations for girls and 0.41 standard deviations for boys , reducing the gender gap in average test scores by a third. Researchers noted that distance from school, a barrier that the intervention helped overcome, was gender specific since cultural norms in Afghanistan restricted women’s and girls’ ability to travel long distances.
The notion that gender might influence the impacts of programs aligns with a finding from J-PAL’s literature review on women’s agency, which highlighted that gender norms might moderate or even block program impacts on women and girls (regardless of whether gender dynamics are taken into account in the design of a program). Thus, in the presence of gender-specific barriers, gender-neutral programs to improve learning may not benefit girls without gender-specific targets and outcomes . As a best practice, policymakers should consider the role that gender plays when designing programs to improve learning.
In general, programs to improve student learning had similar impacts on girls and boys. Although a majority of the 54 studies that reported differences in learning outcome by gender found a larger increase in test scores—measured in standard deviations—for girls, the gender difference in program impact was statistically insignificant in most (44 out of 54) studies that reported it. For example, a technology-aided personalized education intervention in India had a larger impact on girls’ math and language test scores: the program improved girls’ math scores by 0.47 standard deviations and boys’ math scores by 0.34 standard deviations [9]. However, these gender differences in impact were statistically insignificant. Similarly, creating private schools in villages in Pakistan increased students’ aggregate test scores by 0.63 standard deviations [10]. While boys’ test scores improved by 0.6 standard deviations and girls’ test scores improved by 0.66 standard deviations, this difference was statistically insignificant.
In some cases, the number of participants in the study may have been too small to detect statistically significant gender differences in program impact. Most studies also found that the magnitude of the gender difference in impact was relatively small: only eight studies found that the magnitude of the impact on boys was at least twice that on girls, in at least one test.
Although most studies did not report whether gender gaps in learning existed before program implementation, similar impacts on boys and girls might lead to the persistence of existing gender gaps in learning. For example, in Peru, an individualized multi-step program to teach math to preschoolers had gender-neutral impacts, and the program failed to close an existing gender gap in learning. This was despite the fact that, according to researchers’ observations, teachers adopted a more gender-neutral approach to teaching by, for example, including boys and girls equally in all activities [11]. Thus, in settings where gender gaps in learning exist before program implementation, gender-neutral impacts are not sufficient to bridge existing gaps and instead lead to their persistence. Policymakers should take into account existing gender gaps in learning while determining whether a gender-neutral impact on learning is a desirable goal.
Although most programs had gender-neutral impacts, in cases where the program’s impacts were different by gender, girls often benefited more. Nine out of 54 studies found statistically significant differences in program impacts between girls and boys [3][6][7][8][12][13][14][15][16]. In six out of these nine studies, girls benefited more than boys. For example, in Uganda, an innovative teaching intervention consisting of a five-step approach to reading instruction for early grade children impacted learning for girls more than it did for boys, closing a gender gap in test scores[12]. The program increased oral literacy scores by 0.14 and 0.22 standard deviations for boys and girls, respectively, closing an existing gender gap of less than 0.1 standard deviations. However, the program had gender-neutral effects on written literacy and numeracy scores. In Tanzania, combining unconditional grants to schools with teacher incentives based on student performance had positive impacts on all students but impacted disadvantaged students, such as girls and students with lower initial test scores, more [14]. The combined intervention impacted girls’ composite test scores (math and language) by 0.10 standard deviations more than boys’ scores. Similarly, a computer-assisted learning program in China improved test scores more for girls than boys in the third grade, but the gender differences disappeared in the long term. Further, the program did not have different impacts by gender among fifth-grade students [13].
To elucidate gender differences in the impact of programs designed to improve learning, studies should report gender differences more consistently. Overall, many studies do not consistently report gender disaggregated results, making it more difficult to learn which programs are most effective at promoting equal learning opportunities for girls. Only half of all studies that measured learning outcomes initially analyzed for this policy insight also reported gender disaggregated results. Most studies did not report whether a gender gap in learning existed prior to program implementation or if the program created, exacerbated, or helped overcome existing gender gaps. Researchers and policymakers implementing interventions to improve learning should track progress by gender consistently in order to identify situations where only one gender is benefiting and to modify the intervention design to prevent either gender from being left behind. In contexts where there are gender gaps in learning, policymakers should design interventions such that they benefit the disadvantaged gender more to help overcome the gender gap.
Abdul Latif Jameel Poverty Action Lab (J-PAL). 2022. "Improving student learning: impacts by gender." J-PAL Policy Insights. Last modified February 2022.
World Bank. 2017. “World Development Report 2018: Learning to Realize Education's Promise.”
Abdul Latif Jameel Poverty Action Lab (J-PAL). 2018. "Increasing student enrollment and attendance: impacts by gender." J-PAL Policy Insights. Last modified February 2019. doi: https://doi.org/10.31485/pi.2262.2018
Hahn, Youjin, Asadul Islam, Eleonora Patacchini, and Yves Zenou. 2020. “Friendship and Female Education: Evidence from a Field Experiment in Bangladeshi Primary Schools.” The Economic Journal 130, no. 627: 740–764. doi: https://doi.org/10.1093/ej/uez064. Research Paper.
Beg, Sabrin A., Adrienne M. Lucas, Waqas Halim, and Umar Saif. “Engaging Teachers with Technology Increased Achievement, Bypassing Teachers Did Not.” American Economic Journal: Economic Policy, Forthcoming. Research Paper.
Abdul Latif Jameel Poverty Action Lab (J-PAL). 2018. "Improving women's representation in politics through gender quotas." J-PAL Policy Insights. Last modified April 2018. https://doi.org/10.31485/2274.ID.2018
Beuermann, Diether W., Emma Näslund-Hadley, Inder J. Ruprah, and Jennelle Thompson. 2013. “The Pedagogy of Science and Environment: Experimental Evidence from Peru.” The Journal of Development Studies 49, no. 5 (March): 719–736. doi: https://doi.org/10.1080/00220388.2012.754432. Research Paper.
Saavedra, Juan E., Emma Näslund-Hadley, and Mariana Alfonso. 2019. “Remedial Inquiry-Based Science Education: Experimental Evidence From Peru.” Educational Evaluation and Policy Analysis 41, no. 4: 483–509. doi: https://dx.doi.org/10.16237371986703102/081. Research Paper.
Burde, Dana and Leigh L. Linden. 2013. “Bringing Education to Afghan Girls: A Randomized Controlled Trial of Village-Based Schools. American Economic Journal: Applied Economics 5, no. 3 (July): 27–40. doi: https://doi.org/10.1257/app.5.3.27. Research Paper | J-PAL Evaluation Summary
Muralidharan, Karthik, Abhijeet Singh, and Alejandro J. Ganimian. 2019. “Disrupting Education? Experimental Evidence on Technology-Aided Instruction in India.” American Economic Review 109, no. 4: 1426–60. doi: https:/doi.org/10.1257/aer.20171112. Research Paper | J-PAL Evaluation Summary.
Barrera-Osorio, Felipe, David S. Blakeslee, Matthew Hoover, Leigh L. Linden, Dhushyanth Raju, and Stephen P. Ryan. 2020. “Delivering Education to the Underserved through a Public-Private Partnership Program in Pakistan.“ The Review of Economics and Statistics (December): 1–47. doi: https://doi.org/10.1162/rest_a_01002. Research Paper | J-PAL Evaluation Summary.
Gallego, Francisco A., Emma Näslund-Hadley, and Mariana Alfonso. 2021. “Changing Pedagogy to Improve Skills in Preschools: Experimental Evidence from Peru.” The World Bank Economic Review 35, no. 1: 261–286. doi: https://doi.org/10.1093/wber/lhz022. Research Paper | J-PAL Evaluation Summary
Lucas, Adrienne M., Patrick J. McEwan, Moses Ngware, and Moses Oketch. 2014. “Improving Early‐Grade Literacy in East Africa: Experimental Evidence from Kenya and Uganda.” Journal of Policy Analysis and Management 33, no. 4: 950–976. doi: https://doi.org/ 10.1002/Pam.21782. Research Paper.
Mo, Di, Linxiu Zhang, Jiafu Wang, Weiming Huang, Yao Shi, Matthew Boswell, and Scott Rozelle. 2015. “Persistence of Learning Gains from Computer Assisted Learning: Experimental Evidence from China.” Journal of Computer Assisted Learning 31, no. 6: 562–581. doi: https://doi.org/10.1111/jcal.12106. Research Paper.
Mbiti, Isaac, Karthik Muralidharan, Mauricio Romero, Youdi Schipper, Constantine Manda, and Rakesh Rajani. 2019. “Inputs, Incentives, and Complementarities in Education: Experimental Evidence from Tanzania.” The Quarterly Journal of Economics 134, no. 3: 1627–1673. doi: https://doi.org/10.1093/qje/qjz010. Research Paper | J-PAL Evaluation Summary
Duflo, Annie, Jessica Kiessel, and Adrienne Lucas. “Experimental Evidence on Alternative Policies to Increase Learning at Scale.” NBER Working Paper #27298. June 2020. doi: https://doi.org/10.3386/w27298. Research Paper | J-PAL Evaluation Summary.
Carneiro, Pedro, Oswald Koussihouèdé, Nathalie Lahire, Costas Meghir, and Corina Mommaerts. 2020. “School Grants and Education Quality: Experimental Evidence from Senegal.” Economica 87, no. 345: 28–51. doi: https://doi.org/10.1111/ecca.12302. Research Paper.
Aker, Jenny C., Christopher Ksoll, and Travis J. Lybbert. 2012. “Can Mobile Phones Improve Learning? Evidence from a Field Experiment in Niger.” American Economic Journal: Applied Economics 4, no. 4: 94–120. doi: https://doi.org/10.1257/app.4.4.94. Research Paper | J-PAL Evaluation Summary.
Akresh, Richard, Damien de Walque, and Harounan Kazianga. “Cash Transfers and Child Schooling: Evidence from a Randomized Evaluation of the Role of Conditionality.” World Bank Policy Research Working Paper #6340. January 2013. Research Paper.
Angrist, Joshua, Eric Bettinger, and Michael Kremer. 2006. “Long-Term Educational Consequences of Secondary School Vouchers: Evidence from Administrative Records in Colombia.” American Economic Review 96, no. 3: 847–862. doi: https://doi.org/10.1257/aer.96.3.847. Research Paper | J-PAL Evaluation Summary.
Araujo, Maria C., Mariano Bosch, and Norbert Schady. “Can Cash Transfers Help Households Escape an Inter-Generational Poverty Trap?“ NBER Working Paper #22670. December 2018. doi: https://doi.org/10.3386/w22670. Research Paper.
Bando, Rosangela, Emma Näslund-Hadley, and Paul Gertler. “Effect of Inquiry and Problem Based Pedagogy on Learning: Evidence from 10 Field Experiments in Four Countries.” NBER Working Paper #26280. September 2019. doi: https://doi.org/10.3386/w26280. Research Paper.
Banerjee, Abhijit V., Shawn Cole, Esther Duflo, and Leigh L. Linden. 2007. “Remedying Education: Evidence from Two Randomized Experiments in India.” Quarterly Journal of Economics 122, no. 3 (August): 1235–1264. https://doi.org/10.1162/qjec.122.3.1235. Research Paper | J-PAL Evaluation Summary.
Barrera-Osorio, Felipe, Kathryn Gonzalez, Francisco Lagos, and David Deming. “Effects, Timing and Heterogeneity of the Provision of Information in Education: An Experimental Evaluation in Colombia.” Working Paper. August 2018. Research Paper | J-PAL Evaluation Summary.
Bassi, Marina, Costas Meghir, and Ana Reynoso. “Education Quality and Teaching Practices.” NBER Working Paper #22719. October 2016. doi: https://doi.org/10.3386/w22719. Research Paper.
Benhassine, Najy, Florencia Devoto, Esther Duflo, Pascaline Dupas, and Victor Pouliquen. 2015. “Turning a Shove into a Nudge? A ‘Labeled Cash Transfer’ for Education.” American Economic Journal: Economic Policy 7, no. 3: 86–125. doi: https://doi.org/10.1257/pol.20130225. Research Paper | J-PAL Evaluation Summary.
Berlinski, Samuel and Matias Busso. 2017. “Challenges in Educational Reform: An Experiment on Active Learning in Mathematics.” Economics Letters 156: 172–175. doi: https://doi.org/10.1016/j.econlet.2017.05.007. Research Paper.
Borkum, Evan, Fang He, and Leigh L. Linden. “The Effects of School Libraries on Language Skills: Evidence from a Randomized Controlled Trial in India.” NBER Working Paper #18183. June 2012. doi: https://doi.org/10.3386/w18183. Research Paper | J-PAL Evaluation Summary.
Cristia, Julian, Pablo Ibarrarán, Santiago Cueto, Ana Santiago, and Eugenio Severín. 2017. “Technology and Child Development: Evidence from the One Laptop per Child Program.” American Economic Journal: Applied Economics 9, no. 3: 295–320. doi: https://doi.org/10.1257/app.20150385. Research Paper.
Das, Jishnu, Stefan Dercon, James Habyarimana, Pramila Krishnan, Karthik Muralidharan, and Venkatesh Sundararaman. 2013. “School Inputs, Household Substitution, and Test Scores.” American Economic Journal: Applied Economics 5, no. 2: 29–57. doi: https://doi.org/10.1257/app.5.2.29. Research Paper.
Duflo, Esther, James Berry, Shobhini Mukerji, and Marc Shotland. 2015. “A Wide Angle View of Learning.” 3ie Impact Evaluation Report. Research Paper | J-PAL Evaluation Summary.
Duflo, Esther, Pascaline Dupas, and Michael Kremer. “The Impact of Free Secondary Education: Experimental Evidence from Ghana.” NBER Working Paper #28937. June 2021. doi: https://doi.org/10.3386/w28937. Research Paper | J-PAL Evaluation Summary.
Duflo, Esther, Rema Hanna, and Stephen P. Ryan. 2012. “Incentives Work: Getting Teachers to Come to School.” American Economic Review 102, no. 4: 1241–1278. doi: https://doi.org/10.1257/aer.102.4.1241. Research Paper | J-PAL Evaluation Summary.
Edmonds, Eric V. and Maheshwor Shrestha. 2014. “You Get What You Pay For: Schooling Incentives and Child Labor.” Journal of Development Economics. 111: 196–211. doi: https://doi.org/10.1016/j.jdeveco.2014.09.005. Research Paper.
Fryer, R. G., Steven D. Levitt, John List, and Sally Sadoff. “Enhancing the Efficacy of Teacher Incentives through Framing: A Field Experiment.” Working Paper. May 2019. Research Paper.
Garcia Moreno, Vicente A., Paul J. Gertler, and Harry A. Patrinos. “School-Based Management and Learning Outcomes: Experimental Evidence from Colima, Mexico.” World Bank Policy Research Working Paper 8874. July 2019. doi: https://doi.org/10.1596/1813-9450-8874. Research Paper | J-PAL Evaluation Summary.
Gilligan, Daniel O., Naureen Karachiwalla, Ibrahim Kasirye, Adrienne M. Lucas, and Derek Neal. 2022. “Educator Incentives and Educational Triage in Rural Primary Schools.” Journal of Human Resources 57, no. 1: 79–111. doi: https://doi.org/10.3386/w24911. Research Paper | J-PAL Evaluation Summary.
Glewwe, Paul, Michael Kremer, and Sylvie Moulin. 2009. “Many Children Left Behind? Textbooks and Test Scores in Kenya.” American Economic Journal: Applied Economics 1, no. 1: 112–135. doi: https://doi.org/10.1257/app.1.1.112. Research Paper | J-PAL Evaluation Summary.
Hirshleifer, Sarojini R. “Incentives for Effort or Outputs? A Field Experiment to Improve Student Performance.” CEGA Working Paper. October 2021. doi: https://doi.org/10.5072/FK23J3JR55. Research Paper.
Ingwersen, Nicholas, Harounan Kazianga, Leigh L. Linden, Arif Mamun, Ali Protik, and Matthew Sloan. “The Long-Term Impacts of Girl-Friendly Schools: Evidence from the Bright School Construction Program in Burkina Faso.” NBER Working Paper #25994. June 2019. doi: https://doi.org/10.3386/w25994. Research Paper.
Li, Tao, Li Han, Linxiu Zhang, and Scott Rozelle. 2014. “Encouraging Classroom Peer Interactions: Evidence from Chinese Migrant Schools.” Journal of Public Economics 111: 29–45. doi: https://doi.org/10.1016/j.jpubeco.2013.12.014. Research Paper.
Linden, Leigh L. “Complement or Substitute?: The Effect of Technology on Student Achievement in India.” Working Paper. June 2008. Research Paper | J-PAL Evaluation Summary.
Linden, Leigh, Felipe Barrera-Osorio, and Country Colombia. “The Use and Misuse of Computers in Education: Evidence from a Randomized Controlled Trial of a Language Arts Program.” World Bank Policy Research Working Paper. March 2009. doi: https://doi.org/10.1596/1813-9450-4836. Research Paper.
Loyalka, Prashant, Chengfang Liu, Yingquan Song, Hongmei Yi, Xiaoting Huang, Jianguo Wei, Linxiu Zhang, Yaojiang Shi, James Chu, and Scott Rozelle. 2013. “Can Information and Counseling Help Students from Poor Rural Areas Go to High School? Evidence from China.” Journal of Comparative Economics 41, no. 4: 1012–1025. doi: https://doi.org/10.1016/j.jce.2013.06.004. Research Paper.
Luo, Renfu, Yaojiang Shi, Linxiu Zhang, Chengfang Liu, Scott Rozelle, Brian Sharbono, Ai Yue, Qiran Zhao, and Reynaldo Martorell. 2012. “Nutrition and Educational Performance in Rural China’s Elementary Schools: Results of a Randomized Control Trial in Shaanxi Province.” Economic Development and Cultural Change 60, no. 4: 735–772. doi: https://doi.org/10.1086/665606. Research Paper.
Malamud, Ofer, Santiago Cueto, Julian Cristia, and Diether W. Beuermann. 2019. “Do Children Benefit from Internet Access? Experimental Evidence from Peru.” Journal of Development Economics 138: 41–56. doi: https://doi.org/10.1016/j.jdeveco.2018.11.005. Research Paper.
Mbiti, Isaac, Mauricio Romero, and Youdi Schipper. “Designing Effective Teacher Performance Pay Programs: Experimental Evidence from Tanzania.” NBER Working Paper #25903. May 2019. doi: https://doi.org/10.3386/w25903. Research Paper | J-PAL Evaluation Summary.
Muralidharan, Karthik and Venkatesh Sundararaman. “Contract Teachers: Experimental Evidence from India.” NBER Working Paper #19440. October 2013. doi: https://doi.org/10.3386/w19440. Research Paper | J-PAL Evaluation Summary.
Muralidharan, Karthik and Venkatesh Sundararaman. 2011. “Teacher Performance Pay: Experimental Evidence from India.” Journal of Political Economy 119, no. 1: 39–77. doi: https://doi.org/10.1086/659655. Research Paper | J-PAL Evaluation Summary.
Muralidharan, Karthik and Venkatesh Sundararaman. 2010. “The Impact of Diagnostic Feedback to Teachers on Student Learning: Experimental Evidence from India.” The Economic Journal 120, no. 546: F187–F203. doi: https://doi.org/10.1111/j.1468-0297.2010.02373.x. Research Paper | J-PAL Evaluation Summary.
Muralidharan, Karthik. “Long-Term Impacts of Teacher Performance Pay: Experimental Evidence from India.” Working Paper. April 2012. Research Paper | J-PAL Evaluation Summary.
Ozier, Owen. 2018. “Exploiting Externalities to Estimate the Long-Term Effects of Early Childhood Deworming.” American Economic Journal: Applied Economics 10, no. 3: 235–362. doi: https://doi.org/10.1257/app.20160183. Research Paper.
Piper, Benjamin, Wendi Ralaingita, Linda Akach, and Simon King. 2016. “Improving Procedural and Conceptual Mathematics Outcomes: Evidence from a Randomised Controlled Trial in Kenya.” Journal of Development Effectiveness 8, no. 3: 404–422. doi: https://doi.org/10.1080/19439342.2016.1149502. Research Paper.
Wong, Ho Lun, Yaojiang Shi, Renfu Luo, Linxiu Zhang, and Scott Rozelle. 2014. “Improving the Health and Education of Elementary Schoolchildren in Rural China: Iron Supplementation versus Nutritional Training for Parents.” Journal of Development Studies 50, no. 4: 502–519. doi: https://doi.org/10.1080/00220388.2013.866223. Research Paper.
Yang, Yihua, Zhang, Linxiu, Zeng, Junxia, Pang, Xiaopeng, Lai, Fang, Rozelle, Scott. 2013. “Computers and the Academic Performance of Elementary School-Aged Girls in China’s Poor Communities.” Computers and Education 60, no. 1 (January), 335–346. doi: https://doi.org/10.1016/J.Compedu.2012.08.011. Research Paper.