Lessons learned in collecting data for government and randomized evaluations
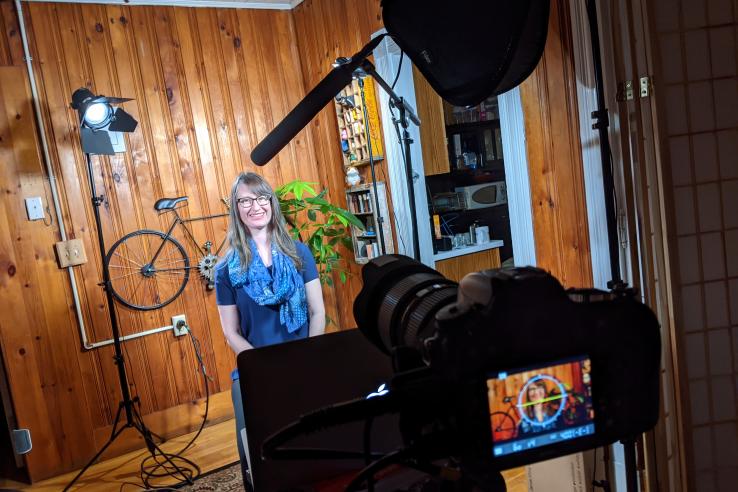
Laura Feeney, Associate Director of Research and Training for J-PAL North America, recounts how her time as an economist at the United States Bureau of Labor Statistics helped prepare her for the realities of implementing randomized evaluations in this blog.
I started my career working as an economist—more specifically an industry analyst—at the US Bureau of Labor Statistics (BLS). I monitored and analyzed pricing trends for various publishing, real estate, and legal industries to contribute to the Producer Price Index (PPI), which is a key indicator of inflation for the US economy.
I left that role to gain tools for statistical analysis, research design, and impact evaluation through a Master’s in Economics. Today, as the associate director of research and training at J-PAL North America, where I lead a team that manages randomized evaluations and teaches research methods through written resources and training programs, I continue to see echoes of my work at the BLS.
At first glance, these roles seem to have very little in common. In terms of subject matter, it’s true: researching book publishing and commercial real estate has not carried over to my work on projects at J-PAL involving subjects such as the take-up of social benefits, mentoring and job readiness programs, and healthcare coordination.
Yet through 3.5 years as an industry analyst, I learned valuable lessons for working with people to obtain data, writing clearly about complex topics, and obtaining and interpreting data that continue to resonate in my work.
To contextualize these lessons, let’s start with a primer on how the PPI works. The PPI measures price changes in the US economy by tracking prices within individual industries, such as book publishing. Every few years, industry analysts assist in drawing a random, representative sample of firms from that industry. Field economists—a role similar to a research associate or enumerator—visit each sampled firm across the country. The field economist identifies individual items or services—for example, particular books—to include in the index. The firm then receives monthly requests from the BLS for updated pricing information on those items. Reporting is completely voluntary, and no compensation is provided.
With this setup in mind, here is what I learned:
People skills are paramount
To repeat: reporting is not required, and there is no financial incentive for firms to participate. The field economist’s and industry analyst's tools for ensuring a high response rate and high quality data are people skills and persuasion.
If a firm had not responded to several months of requests, I would call that firm to try to prompt a response. I avoided taking an aggressive approach (e.g. “why haven’t you answered this form?”), which would trigger defensiveness and likely a refusal to respond in the future. Instead, I phrased questions trying to be helpful, and accepting as much of the responsibility as possible.
My initial message was typically, “I would like to confirm whether the contact information I have on file is correct and whether you have received the form this month.” If they had received the form, I could ask if I could do anything to clarify the purpose or process for responding, and explain the importance of the data.
At J-PAL, this approach of offering assistance to facilitate cooperation, and taking care not to unduly place blame, has served well when working with data providers, partners, and colleagues.
Keep questionnaires simple and instructions clear
Industry analysts research an industry that will be resampled, develop an instrument for sampling particular items from each industry, and provide instructions to field economists for collecting initial data from each firm. But unlike on a J-PAL survey, where researchers and research managers can train and interact directly with survey field teams, industry analysts never interact directly with field economists, and there is little room for piloting and revision once an instrument is in use.
To collect good data in this scenario, I had to anticipate questions and write clear and concise instructions. Similar to many research projects, there are more interesting details that one may wish to ask than are realistically feasible to collect. I learned to narrow down to the questions or characteristics that mattered most, and let the rest go.
This was especially crucial for any forms filled out by firms rather than by the field economists. I knew time and attention would be limited, and a form that was too complex would be thrown away.
At J-PAL, these early lessons continue to apply to survey design, training for enumerators, and even for behavioral nudge interventions. J-PAL condenses these and similar lessons into resources on increasing response rates of mail surveys, survey design, and minimizing measurement error in measurement, and teaches these concepts in our training programs.
Beware lies, damned lies, and statistics
When stripped of their initial (often messy) original context and aggregated into statistics, numbers can dazzle people into undue credulity. Even seasoned researchers familiar with sources of bias and error inherent in primary data collection can be dazzled by administrative data or other data provided by government agencies, as the sources of bias and error may be different or more obscure.
Through written guides, training sessions, and webinars about working with administrative data, I emphasize the importance of digging into how and why data were collected, the incentives of the respondents to report (in)accurately, and any (mis)interpretations the reporter may have had about the purpose of the data request. In doing so, we can begin to have a clearer understanding of the potential sources of bias and error in the data.
This lesson has been reinforced in many experiences throughout the years, but I first learned it as an industry analyst: After receiving confirmation of no price change for a particular item for many months, I became suspicious. In a call with the respondent, I realized they thought I wanted to see no change. I explained that, as an indicator of inflation, we really wanted to understand the true price, and that we understood that it was likely to rise sometimes!
Other respondents may have been concerned that price information would be used and compared with taxes. I emphasized that information reported was completely confidential, was used only for this statistical purpose, and could not be used or shared for any other purpose.
Just note it
Like my current role, work as an industry analyst required writing about complex topics for less technical audiences. I wrote memos to explain large price changes in my indexes, data collection instructions, and in-depth industry summaries. I built expertise in my assigned industries, and learned to recognize which details were most relevant, when I needed to seek more information to truly understand a concept, and what needed emphasis in each type of report.
Of the many tips my supervisor gave to improve my writing, the one I remember most clearly—and that I’ve passed on to many others at J-PAL—is this: “I never want to see you write ‘it is worth noting’ again. Just note it!!”