So, you got a null result. Now what?
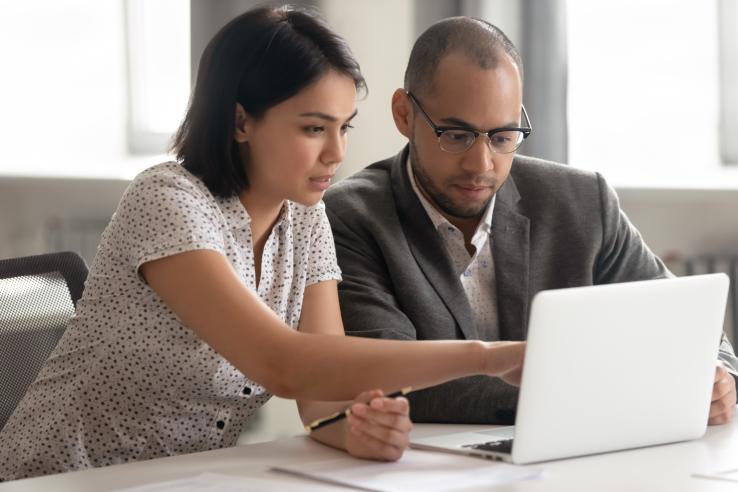
We conduct RCTs to learn. However, the programs we evaluate are often based on strong, evidence-informed theories of change resulting in a hypothesis that the program will have an impact. Therefore, it can be surprising—and disappointing—to run that golden regression only to learn that the impact of the program was not statistically different from zero.
While a null result is likely not what you were hoping for, and while null results can (unfortunately) be more difficult to publish, they can spur just as important insights and policy implications as significant results. However, to learn from a null result, it is necessary to understand the why behind the null. This blog proposes a series of steps that can get you closer to understanding what drives your null result, distinguishing between three explanations: imprecise estimates, implementation failure, and a true lack of impact.
Step 1: Rule out imprecision of the estimates
Establish whether you are dealing with a tightly estimated null effect—a “true zero,” as Dave Evans calls it—or a noisy positive/negative effect. A tightly estimated null effect can be interpreted as “we can be fairly certain that this program did not move the outcome of interest,” (i.e., there is evidence of absence of an effect) whereas an insignificant positive/negative effect can be interpreted as “we cannot really be certain one way or the other,” (i.e., there is absence of evidence of an effect).
The following checks can help you determine whether your null result is a “true zero:”
- Look beyond the stars: A tightly estimated null effect is characterized by a small point estimate and tight confidence intervals around zero. If the effect were at the top of the confidence interval (i.e., the point estimate + 1.96 standard errors), would you have considered the program a success?
- Revisit your power calculations: Your initial power calculations were likely based on a host of assumptions, including the variance of the outcome, take-up rate, rate of attrition, and explanatory power of covariates. Now that you know the realized value of these components, revisit the original power calculations to determine whether any of these assumptions were significantly off. (This exercise is different than conducting ex post power calculations, which is not advised.)
- Rule out data issues: Ideally, data is thoroughly cleaned before it is used for analysis. However, sometimes issues can be missed, which can result in misleading results. It is important to check the data quality before drawing conclusions regardless of whether you got a null result or a significant result.
- Stress-test your null result: Robustness checks test whether the conclusions are robust to alternative specifications. While you most often encounter robustness checks for significant effects, they are equally important when trying to understand null results. For example, robustness checks could include testing for causal effects across different specifications of the outcome variables, or across different subgroups. Don’t place too much weight on significant results—this exercise is about ensuring the robustness of a null result, not changing the initial hypothesis to match the data, or massaging the data to get a significant result.
If your null result is tightly estimated, well-powered, not caused by data issues, and consistent across outcome specifications and subgroups, your result is likely to be a “true zero” and you can move on to the next steps.
If not, consider whether there is anything you can do to improve the data quality or the power of the study. Take a look at J-PAL's resources on data cleaning and management and power.
Step 2: Rule out failure of the implementation
Once you have established that your result is, indeed, a “true zero,” the next step is to establish whether the null result is driven by what evaluation expert Carol Weiss has described as a failure of ideas vs. a failure of implementation.
A good process evaluation can help determine whether your null result is caused by implementation failure:
- Establish that delivery happened as intended: To assess successful implementation, ensure the program was offered and delivered to the intended recipients (i.e., everyone in the treatment group and no one in the control group). For example, within a business skills training program, were the training sessions conducted as planned? Were there any issues during the training and/or with the implementing partner?
- Establish that take-up of and compliance with the program was sufficient to produce an effect: Another necessary condition for program success is that participants in the treatment group take up the program and that people in the comparison group do not. To continue the business skills training example, did enough people from the intervention group participate in the training to expect an effect? Did they attend all the sessions of the training course or just a few?
To answer these questions, look at all implementation or process data you can get your hands on. It can also be valuable to interview implementers and recipients to see how they understood and engaged with the program. Ideally, some of this data has already been collected as part of the ongoing process evaluation, but you might need to go back and ask additional questions.
If the program was delivered as intended and had sufficient take-up, it is likely that the null result is caused by the program not having the hypothesized effect. The next step is to dig deeper into the theory of change to understand why that might be.
If not, your null result might be caused by implementation failure. In this case, it can be difficult to draw conclusions about whether the program would have worked had it been implemented as intended. Instead, consider using what you learned through your process evaluation to improve the program implementation.
Step 3: Understand where the causal chain broke
Even a high-quality theory of change, which is informed by strong evidence, is full of assumptions.
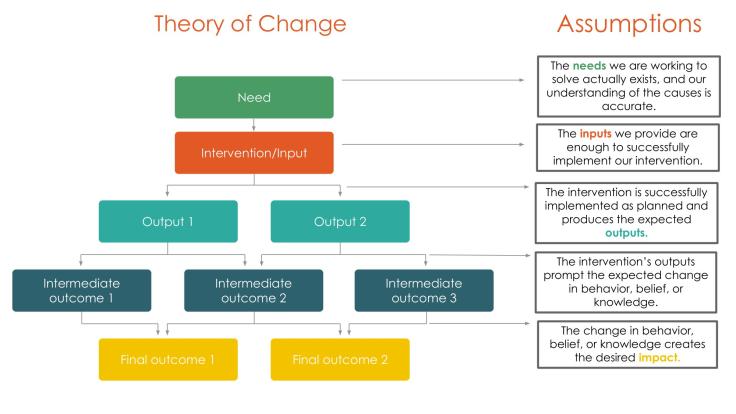
If you ruled out that the null effect was caused by imprecision of the estimate and implementation failure, the next step is to revisit all implicit and explicit assumptions in the theory of change to try to pin down what could have broken the causal chain.
- Assumptions about the need: A good program design starts with a thorough needs assessment. It can sometimes be difficult to establish whether the need being addressed is ultimately the core need (i.e., the binding constraint). For example, a needs assessment might identify that small business owners lack skills in financial management, marketing, and accounting, leading to an informed hypothesis that business skills training could increase profits. However, if the main binding constraint for small businesses is financial, like not having access to credit to expand the business, business training is unlikely to affect profits even if implemented as intended. You can think of this as a failure to identify the core need.
- Assumptions about the intermediate outcomes: Often a theory of change will include a series of intermediate effects that are necessary for the program to be impactful. For business skills training, it is not enough that the recipients attend the training, they also need to learn from it, act on their new information in the intended way, and keep the new practices instead of reverting to old practices after a few months. You can think of this as a failure of the program itself.
- Assumptions about the final outcome: Sometimes even if the intermediate outcomes are successfully impacted, this doesn’t lead to changes in the final outcomes. When this is the case, it can come down to one of the following explanations:
- The program didn’t address a binding constraint, e.g., business skills training programs have been found to be more promising when bundled with financial assistance.
- Implementation of the program had unintended consequences that countered the estimated effect. It could be that the program had positive spillovers to the control group, like if business skills training participants share their new skills with the control group, or that participants in the program substitute away from other equally impactful programs or practices.
- Changing the intermediate outcomes did not lead to a change in the final outcome. This could, for example, happen if some “best business practices” didn’t actually change profits even if fully adopted. You can think of this as a failure of the hypothesis.
Given the nature of assumptions, this step involves forming hypotheses about which assumptions are likely to not have been met. Ideally, you will be able to test these hypotheses, either with existing data or by going back to collect more data (quantitative or qualitative) from implementers, recipients, experts, etc. You can also consider running additional experiments to explicitly test these hypotheses.
Learning from a null result
A general implication of a null result is that the program should not be scaled up, as is, in the given context. However, a null result does not mean you should automatically abandon the program altogether. What you can do with the null result depends on the why of the null:
- A failure of precision of the estimate implies more (sufficiently powered) research is needed on the impact of the program before scaling up
- A failure of implementation implies the implementation model needs to be tweaked and, ideally, evaluated again before scaling up
- A failure of assumptions/hypothesis implies you need to return to the drawing board: The program either needs to be tweaked to take into account what you have learned about the local conditions, or accompanied with another program that addresses the more immediate needs. Alternatively, you should consider another program with a different theory of change
Finally, what you learned about the necessary conditions for program impact can be used—if properly communicated and not filed away in a drawer—to assess the limits to the generalizability of the program and to help design future programs, both in your context and in others. Don't let a null result discourage you; instead, use it as an opportunity to refine and advance your work.