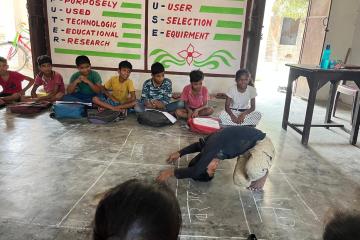
Test, learn, adapt: Maximizing impact through continuous rapid evaluation
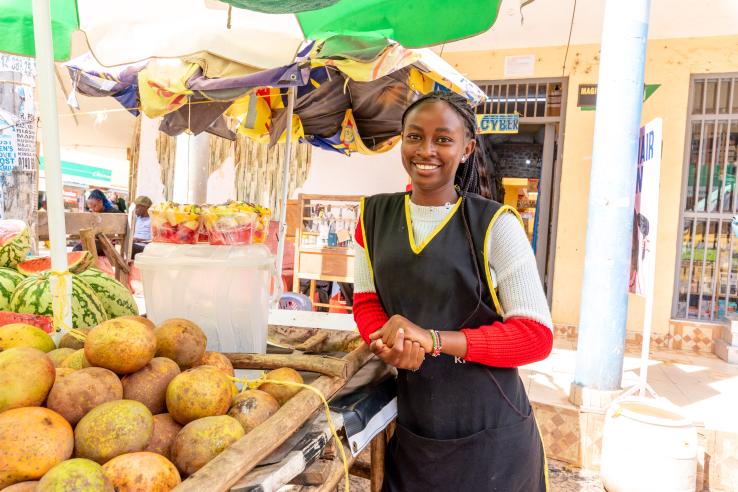
Photo credit: George Mimano
In this guest blog, staff from Educate!, a youth employment organization in East Africa, share how they incorporate learnings from randomized evaluations into their program design and implementation.
There has been an encouraging shift within global development toward evidence-based decision-making over the last two decades. Policymakers and practitioners increasingly use data on effectiveness to assess interventions and many donors require evidence of improved outcomes before committing resources.
While the use of rigorous evidence to make decisions by both practitioners and donors has increased, using data and evidence to inform program design and development is not commonplace. Evaluation is often considered after programs are already implemented, and in some cases, after they are scaled up to reach wider populations. Some evaluations can take years to return results, meaning insights come too late to make changes during critical stages of program development. Embedding faster or ‘rapid’ evaluation early within the program development process can provide valuable, real-time insights that can lead to greater impact and cost-effectiveness before a program scales.
While testing an early model, learning quickly, and adapting in response to insights can maximize impact, this approach takes hard work to get right. In practice, there are challenges to doing this, especially within international development, notes World Bank economist David McKenzie. It requires teams to invest in capacity building, specifically adopting new systems and tools to collect and analyze data quickly, as well as new ways of working with stakeholders—such as donors— who must provide greater flexibility to support iterative learning.
Chris Blattman, a J-PAL affiliated professor who studies conflict, crime, and poverty says in Why We Fight: The Roots of War and the Paths to Peace:
“Almost every big project I’ve seen started the same way. A mayor, a government ministry, or an aid agency comes up with an idea; they write up a program manual outlining who will get what; they get funding from the central government or an outside donor; their approach gets laid out ahead of time in the grant proposal; they immediately implement at scale, to thousands or tens of thousands of people. A hundred things go wrong. They tweak the rollout to fix the worst problems. But the core design never changes, no matter how flawed.”
Faster learning through rapid evaluation can strengthen model design and delivery
Adopting this approach has been critical at Educate!, a youth employment organization in East Africa, as we set out to create a new livelihoods model to support the millions of youth unable to complete secondary school with the skills to thrive within local economies.
In developing this model, which equips young people with the practical skills and experience needed to start a business, we drew on evidence from external randomized evaluations—a methodology championed by J-PAL within the development sector— to inform our approach.
Evidence from a randomized controlled trial (RCT) on Educate!’s employment and entrepreneurship model delivered in Ugandan secondary schools saw significant impacts on business ownership and income as youth were leaving the program. Four years later, this translated into improvements in skills, educational attainment, and gender-related outcomes, such as more egalitarian views about the role women play in households and society, and a reduction in the threat and incidence of intimate partner violence. We also applied insights from an RCT of a three-week bootcamp for Ugandan secondary school students that showed graduates earned around a quarter to a third more income than peers in a control group. The model and study were designed and evaluated by researchers at UC Berkeley and implemented by Innovations for Poverty Action Uganda with support from Educate!. These findings offered valuable lessons about how Educate! could adapt its curriculum and delivery structures to serve youth who are out of school.
As we embarked on developing a program for a new demographic, we also knew we needed to quickly test our assumptions in real-time. Young people out of school face a unique set of barriers compared to their in-school peers, with girls and young women facing even greater challenges. To build an intervention hyper-tailored to this demographic and ensure we were on track, we needed to address key questions on the model design—and do so quickly.
Inspired by peer organizations with experience in rapid evaluation and lessons from modern product development, which prioritizes rapid testing of interventions, we worked to build Educate!’s own rapid learning and evaluation capabilities.
In 2020, after six months of development, we launched our Rapid Impact Assessment (RIA) system with the aim of generating insights on Educate!’s first model for out-of-school youth. The goal of the system was to enable our team to run continuous or ‘rapid’ learning cycles that link changes in program design and delivery to immediate impacts on youth—making critical connections between model design, delivery, and outcomes for youth. Where an externally-run RCT examining medium and long-term impact can often take years to return results, an internal RIA system can provide rapid insights on how outcomes shift in a few months, answering the critical question, “Is this working the way we intend?” Over time, the RIA system has become a platform to support randomized evaluations, A/B tests, and hypothesis testing, allowing Educate! to increase the rigor of evaluation as a model develops the stability and size to support such tests.
To build Educate!’s rapid learning capabilities, we oriented our processes and systems toward agile evaluation methodologies:
-
Building internal capacity for rapid evaluation: We built Educate!’s capacity to collect and analyze data in rapid cycles in parallel with the delivery of the program. Multiple teams engage in continuous learning ‘sprints’ to examine how changes to program design and delivery lead to meaningful changes for youth, while a dedicated evaluation team continuously works to collect data, refine survey tools, and analyze and share results internally.
-
Adopting agile learning methodologies to empower teams: Teams must be empowered with insights for agile decision-making and swift course correction. Change rarely happens in leaps and bounds but rather in small steps through iteration and improvement, all toward the goal of achieving transformative impact. Trial and error are critical to identifying effective strategies for impact. To facilitate this, we adopted agile learning methodologies, such as embedding pre-/post-evaluations and A/B tests within model development to test competing designs. For example: “What schedule is easiest for young people out of school to participate in, especially young women and girls?”
-
Defining early markers of success: Achieving transformative impact takes time, which poses challenges for assessing an intervention’s effectiveness early on. However, identifying early indicators of success (that lead to intended outcomes in the long term) can indicate that a model is on the right track. Educate!’s teams worked to identify early markers that signal whether youth are developing the skills needed for medium- and long-term outcomes. For example, if our long-term indicator of success is improved economic outcomes for youth, we asked ourselves: “What short-term markers can we measure to quickly assess whether the program is working?” To do this, we looked at the early results from Educate!’s RCT in Ugandan secondary schools, which found that youth, immediately after the program, were more likely to take action to start a business and were earning more income than their peers in the comparison group. These short-term actions or behavior changes could then be associated with those improvements in medium-term outcomes.
The insights and learning loops established by the RIA system are enabling our teams to whittle down a model to its most impactful form, allowing us to learn as we build, iron out kinks, and test important assumptions. For example, we conducted a test in Kenya where we provided capital to help youth start businesses more quickly. However, three to six months later, data revealed participants who did not receive start-up assistance caught up on business ownership, with both groups experiencing income gains. The additional cost of capital did not strengthen the model’s cost-effectiveness. Through this early experimentation, we tested this reasonable assumption and gathered important data points to inform both the impact and cost-effectiveness of the model.
By testing an early model through rapid evaluation, learning from the results, and adapting, practitioners can make important decisions that maximize impact. As a model moves toward scale, we can increase the rigor of the evaluation methodology—progressing from internal outcome tracking to an external RCT. Why invest in a large, high-powered RCT on an immature model, built on untested assumptions? By aligning evaluation methodologies with model maturity, practitioners can take a “fit-for purpose” approach to evaluation.
Restructuring partnerships can create space for iterative learning
If practitioners are to adopt an iterative approach—specifically designed to lead to learning and model pivots—key stakeholders, such as donors, must provide flexibility for experimentation and the capacity to generate continuous data for iterative program development. However, as World Bank economist David McKenzie notes, “It can be really hard to say ‘Give me money and let me tinker for a while.’”
Yet, this is exactly what practitioners need to achieve meaningful impact as well as the outcomes promised to partners. To build cost-effective models, they need both time to figure it out and data-driven insights to uncover what works. Embedding greater flexibility within partnerships is essential for facilitating rapid learning and for demonstrating whether an intervention is actually moving the needle. This approach also acknowledges the hard truth that the world is full of uncertainty, operating environments change, and you can only predict so much upfront within a “proposal.” As a number of development economists have highlighted, the best insights are discovered iteratively through testing, learning, and adapting as new information emerges.
Support for this way of working could come in a variety of forms: tying a program to outcomes while providing greater flexibility around how these outcomes are achieved, expanding the number of funding opportunities that encourage early-stage learning, greater budget flexibility, or even replacing a 20-page project plan requirement with a draft of the design and key hypotheses to test.
As a sector, many of us are committed to building more cost-effective and sustainable programming that creates real impact. To achieve this, we not only need new evaluation tools but also new ways of working together—ensuring we effectively maximize impact and continuously strengthen our solutions.