The elements of a randomized evaluation
Summary
This resource presents a high-level overview of the steps of a randomized evaluation, while showcasing a selection of our teaching and learning tools that were created as part of our online and in-person training activities. Throughout this section, the reader will find written resources, video lectures, and case studies about different aspects of a randomized evaluation, derived from our Evaluating Social Programs course and other trainings. For more of these materials, see our teaching resources.
1. Theory of change
The first step in an evaluation is to revisit the program’s goals and how we expect those goals to be achieved. A theory of change model (sometimes called a logical framework) can help in this process. The theory of change can potentially build on other tools from the monitoring and evaluation framework, such as a needs assessment (which provides a systematic approach to identifying the nature and scope of a social problem) or a program theory assessment (which builds a theory of how the program works and describes the logical steps by which the proposed policy fills the identified need).
Next, the researchers need to determine the precise research question they want to ask. Can their question be answered by testing a causal relationship or hypothesis? Which components can be randomized (e.g., who gets the program or different versions of the program, who delivers the program, or when the program is delivered)? How do the program and theory of change map onto the research question? What data can the researchers collect that will answer their research question?
For more information on theory of change, see our Theory of Change and Measurement lecture slides and case studies. In addition, IPA’s “Guiding Your Program to Build a Theory of Change” outlines the preparatory work needed to guide a team through the process of building a theory of change and what to expect at each stage.
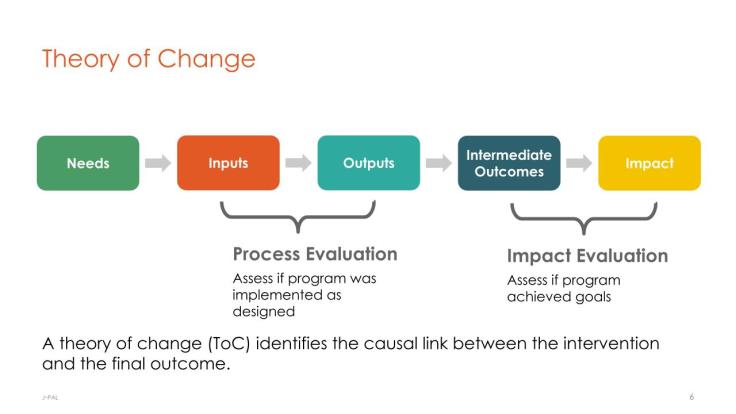
2. Measurement
Measurement in a randomized evaluation typically occurs at two key stages: baseline measurement, often used to gather descriptive statistics on the study sample (such as the average age, household income, or gender composition), and endline measurement, used to estimate the effect of the intervention. Some randomized evaluations may collect data during the middle of the intervention, known as midline data–this is typically used to monitor the implementation of the intervention.
Many randomized evaluations rely on conducting surveys to measure outcomes. Social science researchers often collect innovative and very specific measurements to get at the exact outcomes that the impact evaluation is interested in. For example, researchers can measure the strength of individual preferences for taking on risk or for reciprocating a generous gesture, detailed location using GPS data, information on social networks and financial relationships in a village, quality and quantity of goods purchased and consumed, women’s and girls’ empowerment, and more.
In addition to survey data, the use of administrative data has also become increasingly common. Administrative data in randomized evaluations has enabled researchers to answer questions about a host of subjects at relatively low cost. For instance, J-PAL researchers have answered many important questions about health access and health financing for low-income households in the US using Medicare and Medicaid data. Administrative data is extremely useful to conduct long-term follow-ups on the study sample, and new technologies and the wider availability of big data have enabled researchers to use satellite data to measure deforestation and target cash transfers. For more practical guidance on administrative data, as well as case studies featuring research-data provider partnerships, see J-PAL’s Handbook on Using Administrative Data for Research and Evidence-based Policy.
J-PAL affiliated professor Kelsey Jack explores these concepts in our 2016 Evaluating Social Programs course (video and slides). In addition, J-PAL has technical resources on measurement and survey design, as well as a Repository of measurement and survey design resources by topic. Some evaluations by J-PAL affiliated researchers that use administrative data to measure outcomes can be found below:
3. Deciding on a research design that is ethical, feasible, and scientifically sound
a. Ethics
In some cases, there may be ethical concerns about conducting a randomized evaluation, such as when the intervention is an entitlement, or the implementing partner has the resources to treat the full study sample and it may not be appropriate to select a control group that does not receive the intervention. Design modification may help to address these concerns. It is possible to conduct a randomized evaluation without restricting access to the intervention, for example. As described in Introduction to randomized evaluations, we could randomly select people to receive encouragement to enroll in a program, such as reminder emails or phone calls, without denying any interested participants access. If the implementing partner has the resources to treat the full sample–but does not yet know if the intervention is effective–researchers can use a randomized phase-in design to initially treat part of the sample and learn the intervention effects before extending the treatment to the full sample.
For more details on how research design can ethically solve challenges to randomization, see our Ethics lecture (video and slides), Ethics resource, and J-PAL North America’s guide to Real-World Challenges to Randomization. For information on how to write IRB applications for a research study and to navigate the legal and institutional requirements when working with an IRB see our Institutional review board proposals resource. Information on how J-PAL research projects comply with IRB ethical guidelines can be found in J-PAL’s Research Protocols.
b. Feasibility
In the most basic randomization design, researchers randomize who does and does not receive the program. If that is unfeasible, such as in the above examples due to ethical considerations, researchers can modify the design. For example, researchers may randomize when groups receive a treatment or who delivers the treatment instead of randomizing overall access to the treatment. Note, too, that the cost and effort in conducting surveys and implementing a program will have implications for the design and sample size of a randomized evaluation. These modifications may change the generalizability of the research findings (see below), but preserve the internal validity of the findings due to the randomization. See J-PAL North America’s guide to Real-World Challenges to Randomization and their Solutions, our Randomization resource, or our How to Randomize lecture for more information.
c. Choosing the sample size
Part of ensuring an evaluation is statistically sound is ensuring it has statistical power. The statistical power of an evaluation reflects how likely we are to detect any changes in an outcome of interest if the program truly has an impact. Researchers conduct power calculations to inform decisions such as how many units to include in the study, the proportion of units allocated to each group, and at which level to randomize (e.g., student versus class of students versus entire school). Power calculations can be conducted to ensure researchers have the necessary sample size to detect the main effect of a program, as well as to detect if the program effect differs between treatment arms or for different subgroups of the population. Evaluators who aim to examine impacts for different groups will need to plan sample sizes with adequate power for detecting impacts on these groups. See former Executive Director Rachel Glennerster discuss power calculations in our Power and Sample Size lecture and exercise. In addition, J-PAL has a resource and guide on conducting power calculations.
d. Threats to internal validity
Like all evaluation methodologies, randomized evaluations face threats to their internal validity, including spillovers, attrition, and partial non-compliance. To ensure the study remains statistically sound, researchers can design the intervention and data collection procedures to minimize or measure such threats. For example, researchers who are concerned with spillovers can randomize at a higher level (e.g., the school level versus student level) to avoid spillovers to the control group and measure the full effect in the treatment group, or vary the proportion treated within a village and measure spillovers on those not treated. For a guided exercise in dealing with threats and analysis, see the Threats section of our Threats and Analysis lecture (video, slides, and case study) or J-PAL North America’s Real-World Challenges to Randomization guide.
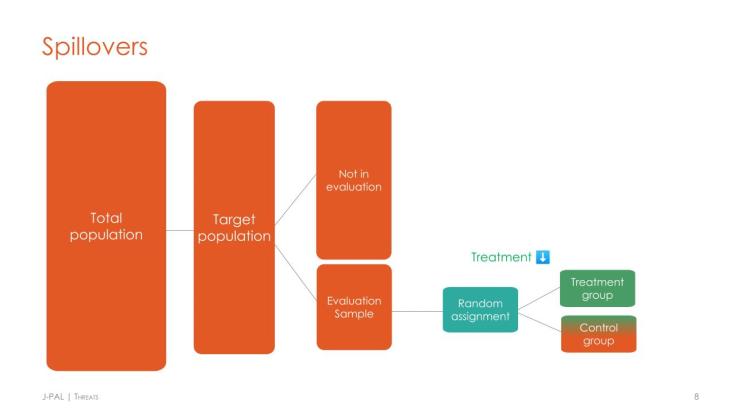
4. Randomization
A key aspect of randomized evaluations, as the name implies, is the random assignment of units into the treatment and comparison groups. Researchers have a range of randomization schemes, ranging from simple randomization, where all units have an equal probability of receiving treatment, to the more complex stratified randomization, where units are partitioned into groups (called blocks or strata), then randomized within the stratum (for example, a study stratifying on gender would randomize within the men in the sample, and, separately, randomize within the women in the sample). An introduction to the different types of randomization can be found in our How to Randomize lecture. For more information on randomization–including when and why stratified randomization is necessary, as well as sample code to conduct randomization–see our Randomization resource.
5. Data analysis
At its simplest, the analysis of a randomized evaluation uses endline data to compare the average outcome of the treatment group to the average outcome of the comparison group after the intervention. This difference represents the program’s impact. To determine whether this impact is statistically significant, researchers can test the difference in the groups’ average outcomes (i.e., test for equality of means) using a simple t-test. One of the many benefits of randomized evaluations is that the impact can be measured without advanced statistical techniques. However, more complicated analyses can also be performed, such as regressions that increase precision by controlling for characteristics of the study population that might be correlated with outcomes.
An introduction to analysis, including analysis in the presence of threats to internal validity, can be found in the Analysis section of our Threats and Analysis lecture (see also the accompanying slides and case study). A more technical resource covering topics such as subgroup analysis, multiple hypothesis testing, and the different types of treatment effects, can be found in our Data analysis resource, which includes additional example code.
6. Cost-effectiveness analysis
Calculating the cost-effectiveness of a program—for instance, dollars spent per additional day of student attendance at school caused by the treatment—can offer insights into which programs are likely to provide the greatest value for money. Cost-effectiveness analysis (CEA) summarizes complex programs in terms of a simple ratio of costs to impacts and allows us to use this common measure to compare programs across time and location. CEA may not, by itself, provide sufficient information to inform all policy or investment decisions, but it can be a useful starting point for choosing between different programs that aim to achieve the same outcome. To conduct CEA, you need two pieces of data: an estimate of the program’s impact and the cost of the program. In order to help other organizations conduct this type of analysis, J-PAL has developed costing guidelines and templates.
7. Generalizability
Unlike internal validity, which a well-conducted randomized evaluation can provide, external validity, or generalizability, is more difficult to obtain. J-PAL has developed a practical framework for addressing the generalizability puzzle, which breaks down the big question of “will this program work in a different context?” into a series of smaller questions rooted in the theory behind a program. For more information, see our lecture (video and slides) or article on the generalizability framework.
Work by J-PAL affiliated professors explores the challenges in drawing conclusions from a localized randomized evaluation through a case study of the Teaching at the Right Level (TaRL) program (Banerjee et al 2017). This program has been tested in different contexts, with different populations, under different implementation models, and through different implementing partners in order to test mechanisms and begin to answer questions on when results generalize. The size of the study population may influence the generalizability of results (Muralidharan & Niehaus 2017). See J-PAL’s Evidence to Policy page for an exploration of how evidence from one context can inform policies in other contexts.
Researchers can further shed light on questions on the external validity and generalizability of results by publishing data from their evaluations. Over the last decade, the number of funders, journals, and research organizations that have adopted data-sharing policies has increased considerably. When the American Economic Association adopted its first policy in 2005, it was among the first academic journals in the social sciences to require the publication of data alongside the research paper. Today, many top academic journals in economics and the social sciences require data to be published. Similarly, many foundations and government institutions, such as the Bill and Melinda Gates Foundation,1 the National Science Foundation,2 and the National Institutes of Health, have data publication policies. J-PAL, as both a funder and an organization that conducts research, adopted a data publication policy in 2015 that applies to all research projects that we fund or implement.3 To facilitate data publication, J-PAL created guides to data de-identification and publication to help research teams think about the steps involved in publishing research data.
Last updated September 2023.
These resources are a collaborative effort. If you notice a bug or have a suggestion for additional content, please fill out this form.
We thank Rohit Naimpally and Evan Williams for helpful comments. All errors are our own.
References
Banerjee, Abhijit, Rukmini Banerji, James Berry, Esther Duflo, Harini Kannan, Shobhini Mukerji, Marc Shotland, and Michael Walton. 2017. "From proof of concept to scalable policies: Challenges and solutions, with an application." Journal of Economic Perspectives 31, no. 4: 73-102. https://pubs.aeaweb.org/doi/pdf/10.1257/jep.31.4.73
Muralidharan, Karthik, and Paul Niehaus. 2017. "Experimentation at scale." Journal of Economic Perspectives 31, no. 4 (2017): 103-124. https://www.aeaweb.org/articles?id=10.1257/jep.31.4.103