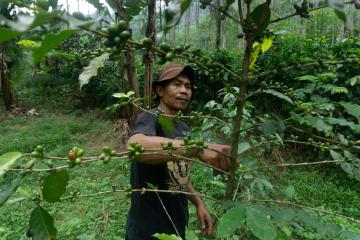
New resource: Incorporating remote sensing data into randomized evaluations
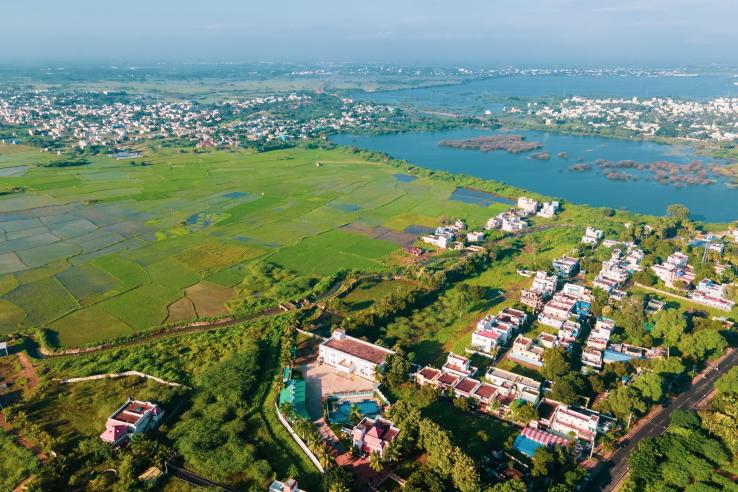
A growing number of economists are incorporating remotely sensed (RS) data—satellite data in particular—into their studies. For randomized evaluations, remote data collection offers alluring possibilities: lower data collection costs, a longer time series of data both before and after an intervention, geographic spillovers, and more. However, the initial allure may obscure some practical challenges.
In a new set of guidelines, J-PAL affiliated professor and Co-Chair of J-PAL’s King Climate Action Initiative Kelsey Jack (University of California, Santa Barbara) and Kendra Walker (University of California, Santa Barbara)—with contributions from J-PAL affiliated professors Jenny Aker, Seema Jayachandran, Namrata Kala, and Rohini Pande, along with Ben Moscona, Sebastien Costedoat, Carlos Muñoz Brenes, Tamma Carleton, Robert Heilmayr, and Johanne Pelletier—outline some of the opportunities and challenges associated with using remote sensing data in randomized evaluations. The guidelines provide resources and recommendations to help social scientists, practitioners, and their collaborators effectively leverage RS data in their evaluations.
Remote sensing refers to collecting data from a distance. Examples of sensors used to collect RS data include on-site monitors, manned or unmanned aircraft systems, and satellites. Predictive models and machine learning methods are often used to interpret raw RS data, such as classifying a satellite image of a piece of land as forested or not. This interpretation stage is typically necessary to allow the researcher to use the data for their analysis.
The guidelines are organized around three main reasons that researchers conducting randomized evaluations might wish to include RS data: (1) increase statistical power, (2) measure different or more objective outcomes and (3) extend analysis to more time periods or locations. For example, RS data may be especially useful when evaluating environmental or agricultural interventions, such as forest cover, crop yields, land use, wildfire smoke and pollution concentrations, since environmental outcomes are often difficult to measure through surveys alone.
While the use of RS data in impact or program evaluation is not new, using RS data in randomized evaluations presents both new challenges and opportunities. Most notably, because randomized evaluations typically involve a substantial amount of researcher discretion over design decisions and primary data collection, researchers can tailor their sample, collect primary data, and interpret RS data to make the most of this new and exciting data source.
The guidelines are structured around the three primary motivations for incorporating RS data into a randomized evaluation and use case studies from Jack’s own experiences using RS data to evaluate the impact of rainwater harvesting techniques in Niger and of payments for ecosystem services on crop burning in India. In this blog, we will briefly highlight selected challenges associated with each of these motivations and examples of how to avoid common pitfalls.
Using remote sensing data to increase statistical power
The larger a study’s sample size, the more likely that the researcher will be able to detect the effect of an intervention if it exists. However, researchers often face logistical or financial constraints that make it difficult to collect primary data for a large number of participants. RS data can help predict outcomes for study participants not included in a survey or other primary data collection, making it possible to include more observations in the study, thereby increasing statistical power.
While RS data may be used to increase sample size, it also introduces a new source of measurement error since outcomes are typically predicted. If the error is sufficiently large, statistical power may not improve much relative to analysis using the smaller set of primary data. For example, if field observations of crop types are used to train a prediction model that achieves only 60 percent accuracy with the RS data, researchers may be better off just running regressions with the field observations. Non-classical measurement error, particularly if it is correlated with treatment, may introduce new forms of bias. For example, if the crop type observations can only be obtained in the treatment group, and treatment affects crop choices, then the model may be systematically more accurate in predicting outcomes in the treatment group than in the control.
Increasing the number or quality of outcomes measured
There are cases where RS data may be more objective, accurate, or inexpensive than primary data collected through surveys. However, some primary data will usually be necessary to calibrate or train the RS model. Raw RS data can be difficult to make sense of without primary data to compare it to. Therefore, designing appropriate primary data collection is important, and may differ from what would be collected in the RCT if no RS data were involved.
One key consideration is linking the relevant unit of intervention in the RCT to the RS data. For example, if agricultural outcomes are of interest, then the researcher needs to know the spatial location of both treatment and control fields. Measurement error will be considerably higher if only geographic points—rather than field perimeters—are collected. Notably, spatial locations must be collected for both treatment and control fields.
RS data may be particularly useful when outcomes are difficult to measure through standard survey-based techniques. For example, illegal activities, such as (in some settings) deforestation or crop residue burning, may be susceptible to substantial reporting error in surveys, but could be more accurately measured with RS data—providing adequate primary data can be collected, of course. Where primary data for training a model cannot be obtained from ground-based methods such as surveys or spot checks (for example, in a conflict zone), a small sample of very high-resolution satellite imagery may provide an alternative approach to constructing a dataset for training or calibrating the RS model.
Extending measurement to locations or time periods outside of the main study sample
Researchers may also want to use RS data to examine the impacts of an intervention outside of the original time period or sample of the evaluation, but as with any statistical analysis, care must be taken when conducting out-of-sample analysis.
First, out-of-sample extrapolation requires an assumption that the relationship between primary (training) data and RS data is the same between the original sample and the extended sample. For example, a land use model trained on data from a set of villages in the original evaluation may or may not perform well for a larger sample of villages that may have been affected by spillovers.
Similarly, the same land use model trained at a single point in time may be poorly suited to predicting the evolution of land use into the future as a result of the treatment. There will almost always be some differences in background characteristics—weather patterns, economic conditions, landscape, etc.—between the main study sample or time period and the extended sample which may cause a model from the original sample to interpret new RS data inaccurately (referred to as “model drift”).
Collecting new primary data for the extended sample and recalibrating the RS model can help with both accuracy and interpretation. If researchers can identify potential opportunities to use RS data to measure spillovers or long-run effects early on, they can design the initial evaluation to make measuring these outcomes easier down the line.
Takeaways
Incorporating remote sensing data into randomized evaluations has tremendous potential to measure outcomes that would otherwise be difficult or expensive to study with traditional surveys and may be especially useful for evaluating environmental interventions that require physical measurements like land cover. However, RS data are not a panacea and researchers need to take these considerations into account from the time they start designing their evaluations to determine whether and how to use RS data.
For more thorough guidance, additional practical considerations, and examples, check out the guidelines here.
Related Content
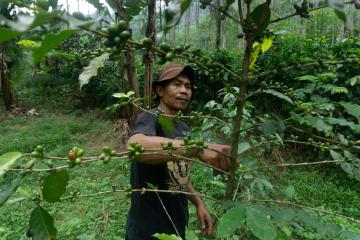
Growing evidence-informed climate policy: Five scaling stories from the King Climate Action Initiative
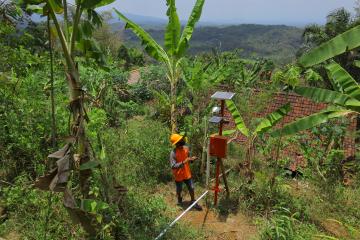
Of satellites and surveys: How innovative data collection can speed up climate action
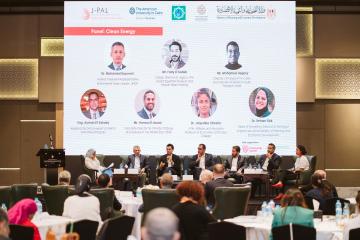