How do we achieve affordable, quality health care? Follow the evidence.
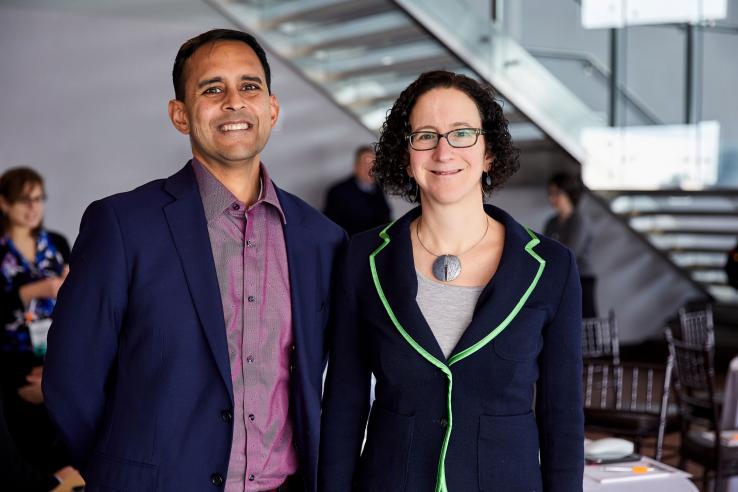
This guest post from Darshak Sanghavi of OptumLabs was originally published on the J-PAL blog on December 6, 2018. Interested in staying up to date with J-PAL North America and the Health Care Delivery Initiative? Sign up for our monthly newsletters here.
As a pediatrician, a health policy director, and now as a chief medical officer, I’ve dedicated my career to improving health outcomes. Through this journey, I’ve been continually inspired by new ways to improve health at scale, and by the important role that rigorous evidence and partnerships can play to improve population health.
In 2016 I worked within the Center for Medicare and Medicaid Services (CMS) Innovation Center to launch the first ever randomized evaluation of a major federal health insurance program in the United States. This is the story of how a commitment to evidence, and a connection by J-PAL North America, played a part to get us there.
As the child of immigrant parents, I dreamed of being a doctor since grammar school. During medical school and a pediatrics residency at Children’s Hospital Boston, my instructors emphasized the importance of patient-doctor relationships to administer quality care at the individual level. But while I studiously developed the technical medical skills necessary to care for individual patients, I understood early on that health care was ultimately practiced at a population level: I was interested in health care improvements that could scale.
Turning point: A new perspective
In 2014, when I was serving as Director of Prevention and Population Health at the Center for Medicare and Medicaid Services (CMS) Innovation Center, I was invited to speak at the J-PAL North America Health Care Delivery Partnership Development Conference about our work. The Center had recently been created through the Affordable Care Act to independently evaluate the impacts of health innovations on cost savings and health outcomes. We were still figuring out strategies to accurately measure these outcomes in systems as large and complex as Medicare and Medicaid.
At the conference I was introduced to Kate Baicker, a leading scholar in the economic analysis of health policy and a J-PAL affiliated researcher. Kate asked me why I wasn’t considering randomization in my evaluations at CMS. My instinct was to think first of the barriers: operational challenges, complex implementation, and more.
And yet, she had a point.
At the time of the J-PAL conference in 2014, CMS had spent billions of dollars on new health service delivery and payment models, but many projects had trouble clearing the bar of evidence for national expansion. We didn’t know for sure whether many programs were improving health outcomes for patients.
A lightbulb went off.
Randomized evaluations may not be possible in every CMS program. But they were certainly possible in some. If CMS could conduct a randomized evaluation, instead of using another evaluation approach, perhaps we could more effectively measure a program’s impact.
Yet even as I (and others) was becoming convinced of the value of incorporating randomization into our evaluations, I still wasn’t sure if it would be possible.
Through my conversations with Kate, I realized that the types of population health models we were evaluating at CMS focused on influencing behavior at the physician or practice level. That in fact made randomization feasible because we would be able to randomly assign physicians and practices to either a treatment or comparison group.
Meeting with Kate at the J-PAL conference was a turning point in my thinking around the importance of randomization and rigorous evidence, and my ongoing conversations with Kate helped answer many questions.
Testing and innovating
Shortly thereafter, an opportunity arose to put these learnings to work. Heart attacks are a leading cause of death in the United States, and we thought hard about how best to test an intervention.
My team began developing the Million Hearts Cardiovascular Risk Reduction Model, which aimed to prevent one million heart attacks and strokes. The model featured a tool that enables doctors to use predictive modeling to assess a patient’s heart attack risk, generate an individualized risk score, then work with the patient to develop an individualized care plan. Doctors receive bonus pay for reducing the absolute risk of heart disease or stroke among their high-risk patients.
In order to conduct a randomized evaluation, we needed hundreds of practices to sign up in order to have a large enough sample size to analyze the program’s impact. To do this, we lowered barriers to entry through a simple five-minute online sign-up process. We also streamlined the contracting process and reserved funds to reward participants in the comparison group as an incentive to remain in the study.
Through national recruiting campaigns, we met our target, enrolling hundreds of practices across rural and urban locations in all fifty states. The model launched in early 2017 and our evaluation, while ongoing, is already delivering useful results.
This early success allowed us to get even more ambitious when designing an evaluation for another CMS model, the Accountable Health Communities.
This project aimed to improve health outcomes and decrease costs by addressing social determinants of health (such as food insecurity and housing instability) by helping patients access screening, referral, and community navigation systems. Although the project is complex, our experience with the Million Hearts model gave us confidence that we could succeed. This evaluation is also ongoing, with results expected in the next few years.
These two models were some of the first randomized evaluations of new payment models from the CMS Innovation Center, and the Million Hearts evaluation was one of the largest randomized evaluations of “paying for prevention” conducted in Medicare and Medicaid.
The future of evidence-based policy in health care
Today, I serve as Chief Medical Officer of OptumLabs. OptumLabs was founded on the belief that collaboration and generating evidence with data is critical to improve health at a systems level. After my work at CMS, I was excited to develop partnerships with organizations to get data into the hands of those who could work with it—and extract actionable insights.
At OptumLabs we have completed more than 110 research projects to date focusing on prominent health issues like the opioid crisis, Alzheimer’s disease, hearing loss, and rising health care costs. By sharing a large de-identified health care dataset with our more than two dozen partners who can act on these data, OptumLabs aims to help organizations improve public health at scale. We try to leverage these partnerships to enhance and facilitate the use of evidence in real-world decision-making.
Today, we face a massive affordability crisis in health care. To achieve affordable, quality care, we must continue to use collaborative, data-driven approaches to generate quality evidence to understand which approaches effectively improve health outcomes and generate cost savings. Through a shared commitment to data and evidence, and partnerships that put data into action, we can help drive evidence-based improvements to scale.
Since this post was written, Dr. Sanghavi has moved to a new post as Chief Medical Officer of UnitedHealthcare Medicare and Retirement.
This is the fifth post in our five-part blog series on the J-PAL North America Health Care Delivery Initiative (HCDI). The first post shares reflections from Amy Finkelstein, J-PAL North America Co-Scientific Director and HCDI Chair, on the role of RCTs in US health care delivery. The second post highlights results from rigorous research on workplace wellness programs and discusses why we should be skeptical of their impact. The third post explores results from an evaluation testing whether a doctor’s race affects black men’s demand for preventive health services.The fourth post features research results from an intervention that sent peer comparison letters to high-prescribers of an antipsychotic drug.