Reducing the cost of lending to low-income borrowers
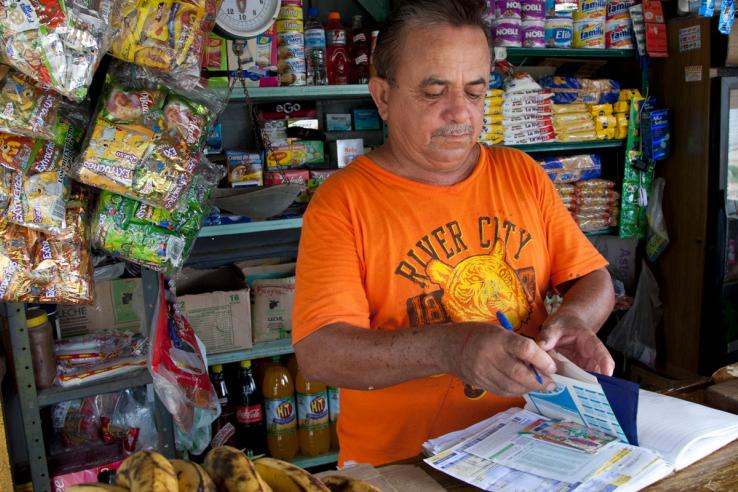
Résumé
Microcredit has made huge strides in the past several decades in lowering the cost and expanding access to credit to millions of borrowers. Whereas local moneylenders were once the source of loans, today formal institutions exist. Yet the price of small loans remains relatively high in many places, as banks pass along high costs of reaching smaller, riskier, or more remote clients in the form of high interest rates (globally, average rates were around 35 percent in 2008, ranging from 17 percent in Sri Lanka to over 80 percent in Uzbekistan) [1]. Low-income households face a choice between taking on costly debt or not borrowing even if a loan could help improve their lives. While barriers remain, several innovations can help reduce costs, including market-wide efforts to generate better information about prospective borrowers, product design modifications that incentivize or enforce repayment, and leveraging technology.
A review of nine randomized evaluations evaluating credit scoring systems, dynamic incentives, and the use of social pressure finds these efforts to reduce costs can improve efficiency and accuracy of bank processes, encourage repayment, and reduce default. These innovations are effective because they generate information that changes decisions of lenders, borrowers, or both. Technology, particularly in the form of digital credit, holds great potential to substantially reduce the costs of providing credit, but impact evaluations are ongoing.
Résultats
Credit scoring reduces transaction costs by helping lenders use borrowers’ histories to make more efficient decisions and improve client selection. Assessing creditworthiness can be cumbersome and costly without reliable information about the credit or financial history of potential bank clients. Credit markets can fail when high costs to assess the riskiness of loan applicants outweigh the financial returns of lending. Credit scoring links a borrower’s behavior to his or her identity, helping lenders use borrowing history or other predictors of future repayment behavior to approve new loans. Credit bureaus and credit scoring software based on verifiable client data increased bank productivity and efficiency in Guatemala and Colombia. In Guatemala, joining a credit bureau helped the lender make larger loans to preexisting clients, end its relationship with underperforming borrowers, and make loans to new borrowers who were better clients overall,with better repayment rates (24 percent reduction in share of loans charged late fees) and 12 percent larger future loan sizes[2]. In Colombia, credit scoring software allowed banks to better allocate loans across borrowers, extending larger loans to less risky borrowers and smaller loans to riskier borrowers [3].
Dynamic incentives, such as the promise of larger loans or the threat of future credit denial, can improve borrowers’ repayment behavior. Lenders can use explicit dynamic incentives to elicit timely repayment and reduce costs. Borrowers whose repayment was linked to preferential future interest rates were 13–21 percent less likely to default on their loan than the average borrower in South Africa. The larger the discount on future loans, the less likely borrowers were to default [4]. Collecting biometric data from borrowers as a screening and enforcement tool increased repayment by 40 percent among the riskiest borrowers in Malawi [5]. Informing or reminding borrowers that their repayment behavior is recorded in a national credit bureau led to improved repayment behavior in Guatemala [2] and Indonesia [6].
Social networks can be leveraged for repayment. Members of the same social networks can encourage repayment from peers. In Peru, individuals receiving loans that were cosigned by friends repaid loans at the same rate as higher-quality borrowers whose loans were cosigned by non-friends. This suggests that friends may have strategies to encourage repayment beyond those available to non-friends or banks [7]. Similarly, while borrowers in South Africa were no better than the bank at identifying reliable borrowers among their peers, they were able to encourage their peers to repay when incentivized to do so; default rates fell from 20 to 10 percent [8]. Banks can leverage some of the power of personal relationships by strengthening relationships between loan officers and clients. In India, borrowers with personal relationship managers at a bank had on average 11 to 13 percent fewer late payments than borrowers with little to no personal contact from the bank, indicating the power of personal relationships[9].
Digital delivery of credit products may bring down costs, but more evidence is needed on impact. In most digital credit products, a mobile phone operator and bank partner to provide small loans directly to borrowers over a mobile money platform. In areas with limited bank infrastructure, digital lending can reduce transaction costs by making disbursements through existing mobile money platforms and agent networks. Yet digital credit also poses new risks: easier access to credit via mobile may lead to overindebtedness and more research is needed to understand the impact of reducing the human interaction that a bank-borrower relationship typically entails. Digital lending also allows banks to leverage nontraditional sources of data (including airtime usage, mobile money activity, or social media accounts) to determine borrowers’ creditworthiness, potentially expanding access to credit to households that would otherwise be screened out. Questions remain about the accuracy of predictions based on nontraditional data, or the potential that nontraditional credit-scoring algorithms may discriminate systematically. A study conducted with a rural bank in the Philippines demonstrates the importance of testing alternative client screening methods. Researchers found that measures of personality traits were not good predictors of default, but actions that revealed honesty were [10].
Abdul Latif Jameel Poverty Action Lab (J-PAL). 2018. "Reducing the cost of lending to low income borrowers." J-PAL Policy Insights. Last modified April 2018. https://doi.org/10.31485/pi.2267.2018