Using Social Networks to Increase Mobile Money Use in Pakistan
In Pakistan, take-up and use of mobile money accounts has been relatively low despite the fact that mobile money is potentially less expensive, more convenient, and can offer a wider range of financial services than many other payment channels. Researchers aimed to conduct a randomized evaluation investigating the impact of incentivizing mobile account holders to refer their friends or relatives to use mobile money on the take-up and sustained usage of mobile money. However, researchers discontinued the evaluation due to difficulties encountered during the implementation.
الموضوع الأساسي
The rapid expansion of mobile phone ownership and use in developing countries represents an opportunity to make everyday payment transactions cheaper, safer, and more convenient for millions of the world’s poor via an alternate payment channel: mobile money. While many believe that these services can improve the lives of the poor, in many countries mobile money uptake still remains low for reasons that may include a lack of knowledge regarding its benefits, low incentives to join, or issues with financial literacy.1 In the context of mobile money and mobile phones, social networks can play a critical role in determining eventual technology adoption. While many studies have sought to identify how to increase the use of mobile money platforms,2 there is a lack of rigorous evidence on the role of social networks in mobile money adoption and active use.
سياق التقييم
In Pakistan, take-up and use of mobile money accounts have been relatively low despite the fact that mobile money may be less expensive, more convenient, and offer a wider range of financial services than many other payment channels. Mobile money has the potential to have a great impact on financial inclusion in Pakistan: while only 8.7 percent of Pakistanis reported having an account registered at a financial institution in 2017, approximately 48 percent of financially excluded adults reported owning a mobile phone.3 Barriers to mobile money adoption include insufficient understanding of services, a limited number of mobile money users in an individual’s social networks, and lack of financial literacy.4
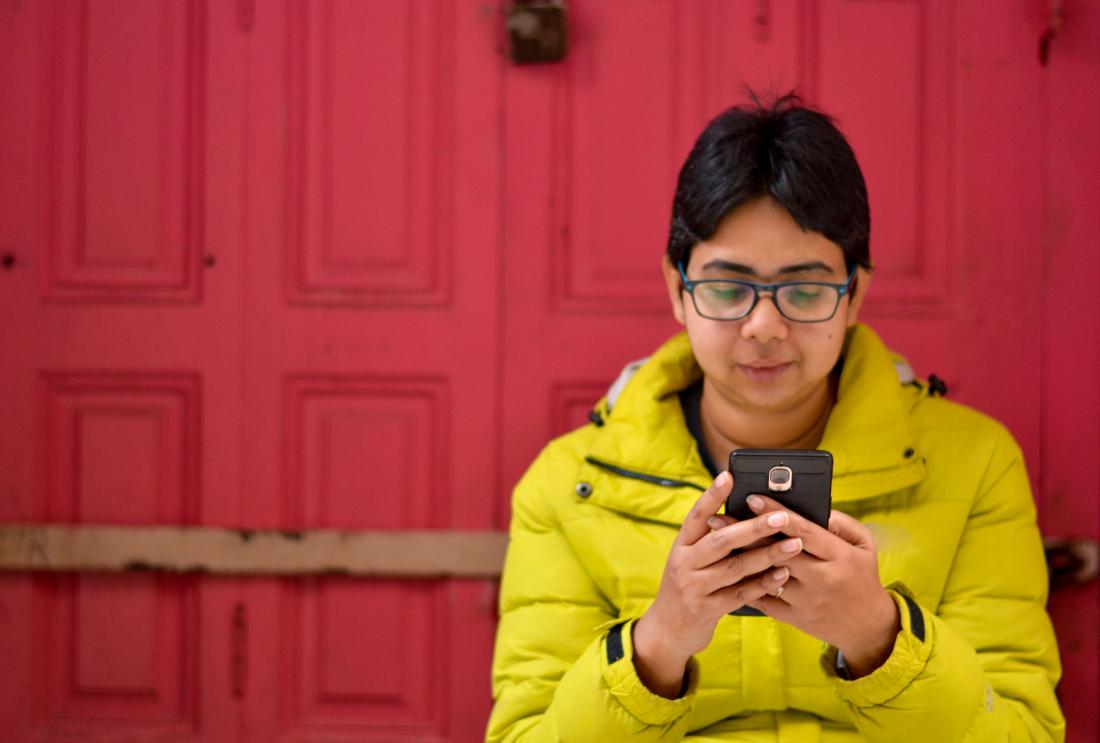
معلومات تفصيلية عن التدخل
Researchers partnered with Pakistan’s leading telecom operator, Telenor Easypaisa, to conduct a randomized evaluation investigating the impact of incentivizing mobile account holders to refer their friends or relatives to the mobile money platform. By leveraging referrals, researchers hoped to identify the channels through which referral-based interventions could be most effective at increasing the take-up and sustained usage of mobile money in Pakistan. Researchers randomly assigned 25,000 active Easypaisa mobile money users to nine groups, each of which was meant to receive a different type of incentive to encourage their contacts to use Easypaisa and/or information about the benefits or how to use the service.
النتائج والدروس المستفادة بشأن السياسات
Researchers discontinued the evaluation due to difficulties encountered during the implementation. Researchers used the available project data to develop a predictive model of mobile money adoption that used billions of mobile phone communications records to understand the behavioral determinants of adoption. They mined the Call Detail Records (CDR) collected by mobile phone operators, which contain detailed metadata on all events that transpire on the mobile phone network, including phone calls, text messages, and mobile money transactions.
Researchers found that the model was only marginally better able to identify active mobile money users (who make at least one transaction per month) than registered mobile money users (who make at least one transaction ever). Results suggest that there is no single set of behavioral features that is likely to consistently predict mobile money adoption and use.
Khan, Muhammad Raza and Joshua E. Blumenstock. "Predictors without Borders: Behavioral Modeling of Product Adoption in Three Developing Countries." Proceedings of the 22nd ACM SIGKDD International Conference on Knowledge Discovery and Data Mining. August 2016. DOI: https://dx.doi.org/10.1145/2939672.2939710