Resources for measuring precise and accurate outcomes in randomized evaluations
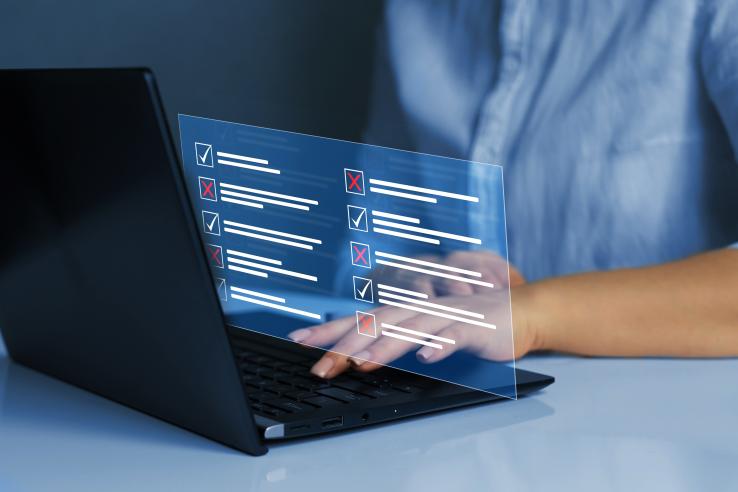
Taking measure(s) of the social sciences
The average sheet of paper weighs 5 grams. Multiply that by 20, add a 226-gram clipboard, and now your 20-page questionnaire is up to a hefty 346 grams.
You’re confident in the accuracy and precision of this measure, but your physicist friend points out that the measure of a gram itself isn’t as stable as you think: if you were an enumerator in the mid-19th century, your questionnaire would be equivalent to the weight of 346 cm3 of water. But water proves unwieldy, so a few decades later your clipboard and package are instead equal to 34.6 percent of a platinum cylinder’s weight.
This reference provides you a century of stability, but now the cylinders you have so diligently compared your survey to vary up to 50 micrograms! So in order to use only universal constants, your questionnaire’s weight is now referenced to a simple combination of Planck’s constant, the speed of light, and the radiation from a cesium atom. (Note: It has been many years since any of the authors of this post took a physics class; we do not stand by the rigor or accuracy of our summary of the source.)
From this sketch it should be clear that physical scientists have put a lot of thought into measurement. From our new repository of measurement and survey design resources, we hope it becomes clear that social scientists have as well.
The repository is a collection of resources that introduce the measurement issues, tools, and innovations in a specific topic or question type. It is a companion piece to our Introduction to measurement and indicators and Survey design pages, compiling resources that discuss and provide guidance on specific issues related to the broader concepts introduced in those resources.
The resources were (and will continue to be) selected from a process that includes a wide search of peer-reviewed journal articles, survey guides, blog posts, and more from social science research organizations; and consultations with experts from J-PAL and its partners with deep experience in the included sectoral areas, such as health and education, and/or with experience conducting randomized evaluations involving collection of related outcome data.
While it aims at introduction over comprehensiveness in any of its individual sections, the repository is still, quite frankly, large and potentially intimidating. In this post, we’ll attempt to ease its navigation by providing a few use cases for research teams at different stages of questionnaire and study design, moving from more general measurement considerations to outcome-specific resources.
Use case 1: Deciding outcomes of interest
Research Team One plans to evaluate a program targeting early childhood health and nutrition. Though the intervention itself and the target group have already been established, the team has not yet decided which outcomes and covariates are of greatest interest.
To help guide this choice, they first look under the “Health indicators” subsection of the Health section. Even a quick survey of the headings gives broad categories for possible relevant outcomes: “Anthropometric data,” “Early childhood development (cognitive),” “Early childhood development (physical),” and “Nutrition” all show promise in guiding thought on outcome choice.
Within the headings, the researchers decide to start with overview resources—those marked “broad overview,” “book”/”book section,” or “blog post” are likely good starting points for getting started on a topic. They settle on the first broad overview, the WHO’s Global reference list of 100 core health Indicators (plus health-related SDGs) to begin the search to identify and narrow down outcomes.
Use case 2: Researching specific indicators
Research Team Two has decided on the concepts that they’ll be measuring, but not yet on the indicators they’ll use to capture them. This team is interested in studying corruption among local government officials; from a couple of the introductory papers in the section, Olken and Pande (2012) and Svensson (2005), they know that there are many different methods for measuring it, from direct observation to evidence in administrative data to survey questions.
This is where a complementary knowledge of the study context is very important: from qualitative work and summary stats from the International Crime Victims Surveys (ICVS) dataset, the team knows that bribery is common among their target population. A quick scan of resource titles and descriptions shows that the subsections “Through direct observation” and “Through survey estimates” contain resources that deal with two methods for measuring bribes. Papers under each subtopic, like Bai et al. (2019) and Olken and Barron (2009), provide introductions to each method, and the examples at the bottom of each subsection show how other teams actually put them into practice.
Use case 3: Learning new methods
Research Team Three is experienced in studying decision-making behaviors around household consumption and investment. However, the bundled program they’re currently evaluating also contains family planning counseling sessions, and they want to extend their study of household decision making to reproductive choices.
The survey guides in the Gender section of the repository, including J-PAL’s “A practical guide to measuring women's and girls' empowerment in impact evaluations,” provide a number of indicators to use and guidance on measuring them. However, depending on the context, women may be reluctant to answer questions on what is often a sensitive topic, and the research team is looking for guidance in ethically asking sensitive questions while reducing the risk of bias.
Again the repository can help: the Sensitive questions section provides an overview of different indirect response survey methods and a few examples of studies where they have been used. Chuang, Dupas, Huillery and Seban (2020) in particular is useful to the team: it compares list randomization and randomized response techniques for collecting data on reproductive decisions. Going further, the subsections on list randomization and randomized response technique introduce the two methods and provide their advantages and limitations with some examples where they have been used (see, e.g., Droitcour et al. (2004) and Blair et al. (2015), respectively).
Moving forward
As with all of our research resources, this repository is a collaborative effort. Though we plan to update it with new resources as they become available, we rely on crowd-sourced material to maintain this page and keep it current with exciting new research areas and methods. If you have any feedback or suggestions of resources to add or remove, please submit them through this form.