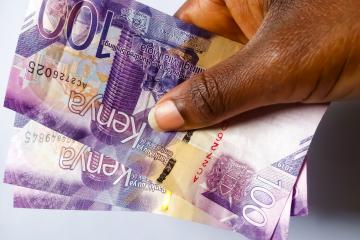
A state-of-the-art, cheap, scalable method to measure child and adult (multilingual) speech in early childhood interventions
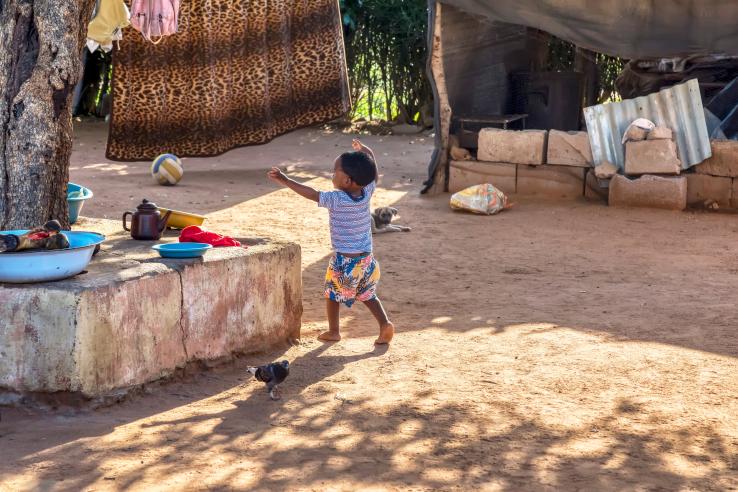
The potential of early childhood development interventions
Early childhood development (ECD) is key to fostering economically prosperous and just societies. A large body of research has established that early childhood interventions generate substantial benefits in terms of educational achievement and lifelong earnings. These interventions have particularly high rates of return for the most disadvantaged within a society and in low-income countries especially ([1]-[5]).
While there is an extensive literature on ECD interventions in high-income countries ([7], [8]) and on intensive (and complex to roll-out) home visit stimulation interventions in low- and middle-countries ([9]), there is, to date, not enough evidence on the effectiveness of early cognition interventions in these countries, and how this varies with, for example, childcare habits, household organization, etc.
ECD interventions can range in focus from nutritional supplementation to more behavioral approaches, including parent information and coaching to increase early cognitive development. One subtype of intervention focuses on boosting child-directed speech from adults in order to enhance human capital development in low-income countries (see e.g., [10], [11]). Our recommendations below are particularly useful for this emergent line of research.
Gathering data on the effectiveness of these interventions is challenging. Rather than relying on survey data or parental reporting, which can be unreliable for reasons outlined below, we propose to turn to child-centered observation methods relying on wearables that capture aspects of the child's and other family members’ behaviors.
Measurement of child (and adult) speech
One obstacle to the collection of evaluative evidence on early childhood interventions is the difficulty of measuring child cognitive development in a consistent way across diverse populations. Few countries have appropriately developed and normalized early childhood cognitive development instruments, and the majority of the world's child population is learning more than one language, making language-based testing particularly challenging.
Language barriers also affect survey-based methods. Some studies rely on behavioral observations, which can be used to measure both the child and those around the child, but these data are very costly to obtain and, as a consequence, sample size is usually too limited to enable statistical inference about policy evaluation. The presence of an observer itself can also bias observations in a way that may be correlated with outcomes of interest.
To measure the behavior of the adults who are sometimes the target of behavioral interventions aimed at increasing child cognitive stimulation, most studies in economics rely on self-reported time use data. This poses several potential limitations, including reporting bias, missing data, and/or significant measurement error. Such measurement and reporting bias may be particularly problematic when correlated with unobservable factors that affect the outcome of interest.
For example, working mothers may have limited time to complete time use logs, despite the fact that they spend a lot of time investing in their children. Both observations and surveys also often assume the mother is the most relevant informant and interactant, but in more than half of the world's societies individuals other than the mother contribute to caring for the child—and in 43 percent, individuals other than the mother are the primary caregiver ([12]).
Child-centered observation methods, relying on wearables that capture at least certain aspects of the child's and others' behaviors, can be a more effective approach. In particular, long-form audio-recordings (lasting 10+ hours) capture vocalizations by both the child and others as the child goes about a typical day. In the 0-4 year range, child vocalization counts correlate with standardized language measures ([13]; [14]), which are the current best predictors of academic achievement ([15]). Others' vocalizations are also recorded, which can be useful in the context of interventions aiming to boost adults' speech to the child ([7]).
Technology options: LENA and USB+VTC
LENA
Economists interested in early childhood development in the context of high-income economies (see e.g., [8]) and, more recently, low- and middle-income economies ([11], [16]) so far rely on a hardware/software combination known as LENA. The LENA Foundation created hardware (a recording device they call DLP, short for digital language processor) as well as a software system, which can only be used together. The software was trained on a diverse sample of English-learning North American children. We refer interested researchers and practitioners to additional information on the LENA hardware/software solution provided here.
The LENA solution is easy to use but expensive (around US $200-400 per device, in addition to per-recording processing costs) and requires reliable and consistent access to electricity and internet, making it an unattractive option for field research with large sample sizes, small budgets, or remote fieldwork conditions.
In addition, the recommended form of processing is by uploading the recording data to the cloud to be processed in LENA servers. Although these are HIPAA compliant, this does mean individuals’ data leaves the country, which may violate local privacy laws.
Instead, we suggest an alternative solution, which we call USB+VTC.
The USB+VTC
Researchers can buy cheap, mass produced, easy-to-use, and easily scalable hardware: audio-recording USBs fitted into a piece of clothing (more information below and here).
Each recording can then be analyzed with open-source software, like the voice type classifier (VTC). The VTC is an end-to-end neural network ([17]) which returns, for the whole recording, the sections (a section consisting of a 10 ms frame) the child wearing the recording device is vocalizing (crying, laughing, or speaking), and when female adults, male adults, or other children are vocalizing. Data can then be analyzed using open-source software (see additional information in “Additional information on USB+VTC”) and/or partnering with researchers familiar with vocalization data processing.
VTC was trained with a combination of various child-centered corpora of children aged 0-4 years exposed to one or more of a variety of languages (including Minn, French, Ju’hoan, Tsimane’, English, and several others, in approximate order of data quantity). Importantly, these corpora included children growing up in multilingual settings, with a wide variety of typological characteristics, both in urban and rural sites. The multi-corpus training improves the generalizability of the network to unseen data sets, making it particularly attractive for development economists working with multilingual environments and under-resourced languages.
As reported in [17], F-score performance on the test set of this multilingual corpus was 77.3% for recognizing the key child. [17] also report on performance for a wholly independent, unseen, test set composed of monolingual English learners' data, for which LENA performance was also available. In that comparative dataset, LENA’s performance for the key child was 54.9%, whereas the VTC scored nearly 15% higher, at 68.7%. The researchers also report better performance than LENA in the other categories (male adult, female adult, other child; see [17] for details).
Other open-source free software has also been developed; for instance, a software called ALICE trained using a multilingual approach can quantify the number of adult words, syllables, and phones ([18]), similarly matching or outperforming LENA.
To summarize, VTC, compared to other available technologies, is:
- Cheaper and more scalable,
- Better adapted to remote fieldwork conditions,
- More precise,
- Better adapted to multilingual environments, with a multilingual (and public) training corpus, and
- More transparent (public training corpus, full replication possible).
LENA technology remains useful in settings that may face fewer resource, language, and logistical constraints. However, VTC and its applications are particularly relevant to development and public economists interested in early childhood development and child human capital as well as anthropologists, psychologist and linguists interested in language development (e.g., [19]).
References
[1] Heckman, J. J., Moon, S. H., Pinto, R., Savelyev, P. A., & Yavitz, A. (2010). The rate of return to the HighScope Perry Preschool Program. Journal of Public Economics, 94(1-2), 114-128.
[2] Currie, J. & Almond, D. (2011). Human capital development before age five. Handbook of Labor Economics, 4, 1315–1486.
[3] Gertler, P., Heckman, J., Pinto R., Zanolini, A., Vermeerch, C., Walker, S., Chang, S. M., & Grantham-McGregor, S. (2014). Labor Market Returns to an Early Childhood Stimulation Intervention in Jamaica, Science, 344(6187), 998-1001.
[4] Busso, M., Cristia, J., Hincapié, D., Messina, J., & Ripani, L. (Eds.). (2017). Learning better: Public policy for skills development. Inter-American Development Bank.
[5] Richter, L. M., Daelmans, B., Lombardi, J., Heymann, J., Boo, F. L., Behrman, J. R., Lu, C., Lucas, J. E., Perez-Escamilla, R., Dua, T., Bhutta, Z. A., Stenberg, K., Gertler, P., Darmstadt, G.L., with the Paper 3 Working Group & Lancet Early Childhood Development Series Steering Committee. (2017). Investing in the foundation of sustainable development: pathways to scale up for early childhood development. The Lancet, 389(10064), 103-118.
[6] Attanasio, O., Meghir, C., Nix, E., & Salvati, F. (2017). Human capital growth and poverty: Evidence from Ethiopia and Peru. Review of Economic Dynamics, 25, 234–259.
[7] Ferjan Ramírez, N., Lytle, S. R., & Kuhl, P. K. (2020). Parent coaching increases conversational turns and advances infant language development. Proceedings of the National Academy of Sciences, 201921653.
[8] Cunha, F., Gerdes, M., & Nihtianova, S., (2021). Language environment and maternal expectations: an evaluation of the Lena Start program. Working Paper, Rice University, July.
[9] Grantham-McGregor, S., Powell, C., Walker, S., & Himes, J. (1991). Nutritional supplementation, psychosocial stimulation, and mental development of stunted children: the Jamaican Study, The Lancet, 338(8758).
[10] Cassar, A., Cristia, A., Delavande, A., Grosjean, P. & Walker, S. (2019). Child human capital production: A field experiment in the Solomon Islands, AEA RCT Registry: AEARCTR-0004116, August 20.
[11] Dupas, P., Jayachandran, S. & Walsh, M. (2018). Promoting Infant-Directed Speech in Ghana. AEA RCT Registry: AEARCTR-0002943. May 15. https://doi.org/10.1257/rct.2943
[12] Barry, H., & Paxson, L. M. (1971). Infancy and early childhood: Cross-cultural studies. Ethnology, 10, 467-508.
[13] Gilkerson, J., Richards, J. A., Warren, S. F., Oller, D. K., Russo, R., & Vohr, B. (2018). Language experience in the second year of life and language outcomes in late childhood. Pediatrics 142(4).
[14] Wang, Y., Williams, R., Dilley, L., Houston, D. M. (2020). A meta-analysis of the predictability of LENA™ automated measures for child language development. Developmental Review 57, 100921.
[15] Pace, A., Alper, R., Burchinal, M. R., Golinkoff, R. M., Hirsh-Pasek, K. (2019). Measuring success: Within and cross-domain predictors of academic and social trajectories in elementary school. Early Childhood Research Quarterly 46, 112–125.
[16] Ma, Y., Jonsson, L., Feng, T., Weisberg, T., Shao, T., Yao, Z., Zhang, D., Dill, S.E., Guo, Y., Zhang, Y. & Friesen, D., (2021). Variations in the home language environment and early language development in rural China. International Journal of Environmental Research and Public Health, 18(5), 2671.
[17] Lavechin, M., Bousbib, R., Bredin, H., Dupoux, E., & Cristia, A. (2020). An open-source voice type classifier for child-centered daylong recordings. Interspeech.
[18] Räsänen, O., Seshadri, S., Lavechin, M., Cristia, A., & Casillas, M. (2020). ALICE: An open-source tool for automatic measurement of phoneme, syllable, and word counts from child-centered daylong recordings. Behavior Research Methods. [preprint, online resource]
[19] Cassar, A., Cristia, A., Grosjean, P. & Walker, S. (2022). It Makes a Village: Allomaternal Care and Cooperation. Working paper, https://papers.ssrn.com/sol3/papers.cfm?abstract_id=4285074.
Additional references:
Video: Clothing options for wearable devices.
Text with full explanations of the technique.
Additional information on USB+VTC
The USB devices generally have a battery life of 15 hours, and cost about 20US$. We recommend using two devices, launched at the same time—in case one of the devices fails, one always has a back-up. The devices can then be recharged by plugging them to a USB charger. This will allow the family to collect several such recordings—for instance for 4 days. The precise storage capacity depends on the brand used. This page provides information on a test comparing several devices, which can be used to find the most suitable USB device. Further information can be found here.
The VTC is an end-to-end neural network, that is, a trained classifier using state-of-the-art neural approaches. Watch a video explanation of neural networks.
Code for VTC. The code is free and open-source. It can also be re-trained.
Code for ALICE. The code is free and open-source. A retrainable Python version is forthcoming.